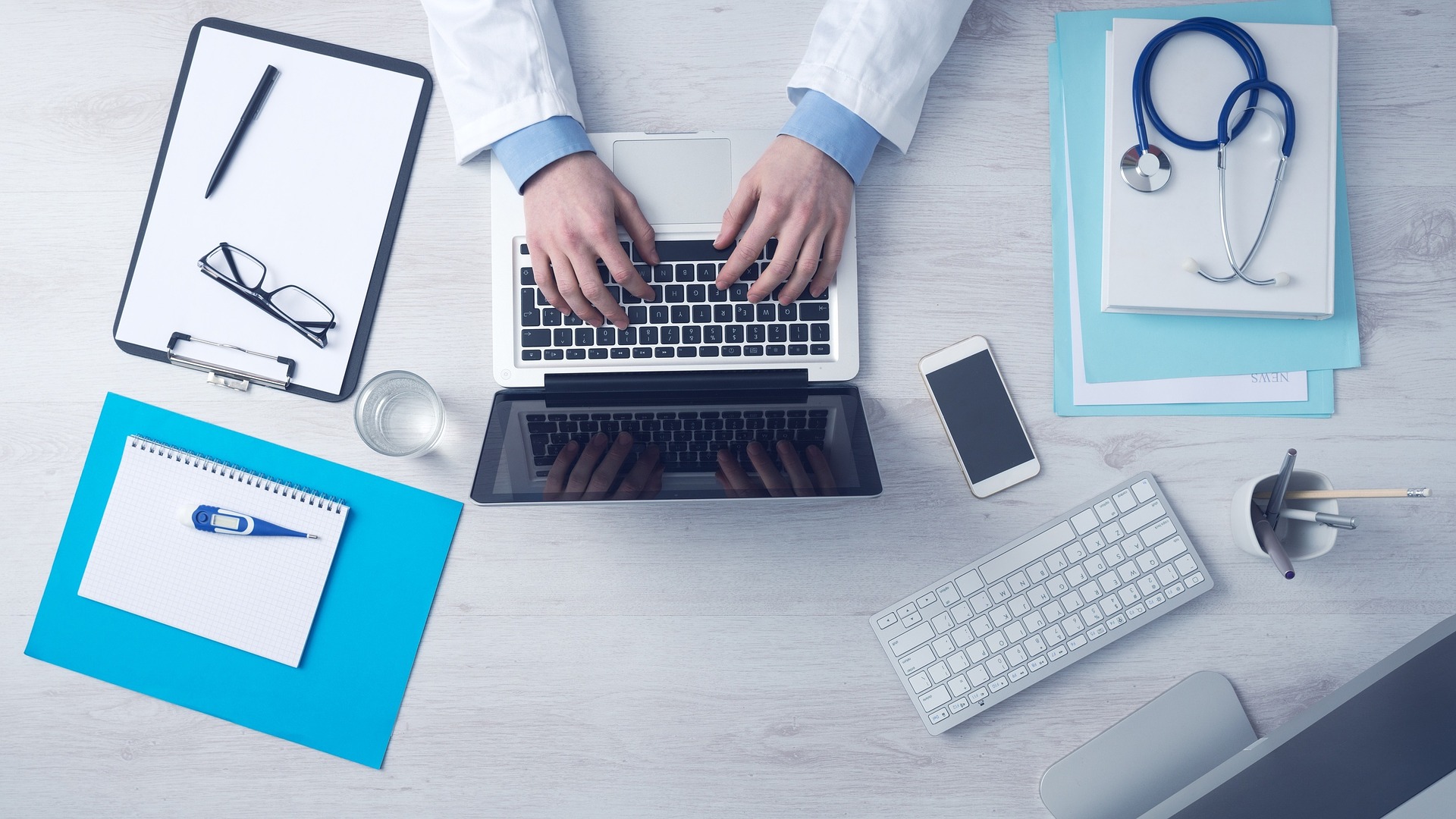
June 4, 2019
4 min read
The Role of AI in Healthcare
Author:
Nick Kartman, Technology evangelist passionate about DevOps, Big Data, ML, and Cloud
AI-powered machines improve human performance in diagnosis of cancer and other diseases
The invention and rise of computers in the 1950s brought along the rise of Computer Science and various types of research related to the topic. Arguably, one of the most prominent inventions was Artificial Intelligence (AI) that was first presented at Dartmouth College in 1955. The first AI was a chess-playing algorithm that “learned” how to play the more it was used.
Since then, AI came a long way as it was used in aviation, research, and medicine. One of the most popular modern applications of AI can be seen in the field of computer vision, or, specifically, in medical imaging and computer-assisted diagnosis (CADe).
CADe, algorithms that analyze images, became an essential part of modern radiology because it allows medical professionals to diagnose various types of cancer and other “scannable” diseases (i.e. glaucoma) more efficiently.
AI vs Doctors
— Bon Ku, MD, MPP (@BonKu) May 31, 2019
"By feeding huge amounts of data from medical imaging into systems called artificial neural networks, researchers can train computers to recognize patterns linked to a specific condition"https://t.co/8cxKSrr6e8 pic.twitter.com/vKRM9lh9nb
The use of CADe can be traced back to the 1990s when the first digital mammography tool was utilized. However, it was not a popular research topic until the most recent decades because of lack of technological advancements and data.
In the early 2010s, however, CADe became more effective and useful because of the invention and use of tissue microarray (TMA), a part of the histological analysis. On top of that, archived histological studies made it possible to use historiographical tissue patterns and computer-aided imagery analysis to make the CADe predictions more accurate.
Both of these are crucial to the improvement of CADe as they allow for the computers to use algorithms to “learn” how to identify and classify various types of cancer.

The more the machine learning algorithm is used, the more data it has, the better it becomes at identifying the disease.
One of the most popular uses of CADe in cancer identification uses histopathological images to analyze the disease. Histopathological images come from the microscopic examination of tissue that carries the most information about the status of the disease.
The images are taken from under the microscope and are optimized using Gabor filter and fast Fourier transformation. After that, images are broken into segments that an algorithm can analyze and determine potentially cancerous cells.
The histopathological procedure is only one way to diagnose cancer at its early stages but it is considered to be the “golden standard” of radiological studies because it carries the most information about the affected tissues.
The use of CADe combined with images from histopathological studies expands opportunities for medical professionals when it comes to diagnosing cancer.

CADe combined with histopathological images makes the diagnosing process easier, more accurate and efficient as it becomes more and more advanced.
However, such a system requires a lot of specific resources which makes it difficult to place CADe in all hospitals. One of the main issues has to do with the hardware as CADe needs a lot of computing power to perform its functions in the most efficient and timely matter.
The more computing power is available to the system, the more efficient it becomes which makes it more useful to the specialists. These problems are expected to be dealt with shortly, as the computing power doubles every two years.
Even though there are some difficulties with implementing CADe, the system could revolutionize the diagnostic field by making the process of diagnosing cancer faster and more accurate.
As of today, only a relatively small number of CADe systems has been produced and released for public use. Their main aim is to aid radiologists by pointing out the specific regions on the images that might need some extra consideration.
The potential of the CADe systems does not stop there as it is possible, in the future, to use CADe for patient management. This would mean that the prescriptions and further treatments would be given based on the computer-generated feedback.
Scientists hope that computers would be able to mimic the human “eye-to-brain” image analysis which would simplify the diagnosis of diseases making it even more efficient.
Indeed, some of the modern companies already made enormous progress in the development of AI systems that support that would improve the CADe and potentially make it more available to the public. For instance, a San Francisco based company called Freenome focuses on developing and improving AI that can recognize cancer and detect it earlier traditional methods.
Alternatively, computer vision can be used for non-invasive detection of visually identifiable diseases and pathologies. For instance, Pr3vent uses AI for accurate screening and processing of infants’ retinal images, which helps identify eye pathologies more efficiently and ensure that children are treated earlier to avoid vision loss.
With companies such as Freenome and Pr3vent researchers and scientists could hope to advance the algorithms to match or exceed the abilities of medical professionals.
Computer vision enables CADe, which has the potential to bring a highly accurate diagnosis of cancer and other diseases to every hospital. The most recent technological advances and their applications show that in the nearest future it would be entirely possible to overcome many of the difficulties that humans faced for hundreds of years.
Looking to empower your organization using computer vision? Reach out to Provectus and discover our AI solutions for business!