Revolutionizing Sleep Through AI-Driven Temperature Recommendations
Sleepme automates real-time bed temperature adjustments and improves sleep quality through AI/ML & IoT technology
Sleepme is a healthcare technology pioneer specializing in sleep management and monitoring solutions. With a focus on enhancing sleep quality, Sleepme has developed state-of-the-art sleep systems that have improved the sleep of over 150,000 satisfied customers. Their flagship products, Dock Pro and Cube, offer personalized temperature control, ranging from 55-115°F, to ensure optimal sleep conditions. Sleepme systems are designed to be user-friendly and highly effective, with features like rapid cooling and warming, scheduled temperature adjustments, and AI-driven real-time sleep tracker insights. Sleepme’s innovative approach to sleep technology has made them a go-to solution for people seeking a cooler, deeper, and more restful sleep.
Challenge
Today, sleep quality is often compromised by factors like stress, fast-paced lifestyles, and suboptimal environmental conditions. Sleepme recognized the need for a real-time, adaptive system that could automatically cool or heat the bed based on room temperature, humidity, heart rate, and sleep cycles, to improve the quality of sleep for its customers. The challenge was to create a machine learning solution that could provide recommendations for bed temperature adjustments, and do so with low latency, in real time, while integrating data from various IoT sensors and sources.
Solution
Sleepme joined forces with Provectus to further develop and improve their machine learning solution for real-time temperature recommendations. The ML model was trained on a comprehensive dataset that included historical sensor readings and user feedback, and utilized AWS services to provide low-latency recommendations in real time. The solution was designed to be highly available and scalable, delivered ready-to-use, to ensure that Sleepme would not need to manage infrastructure but focus on delivering new products and features for their customers.
Outcome
Within weeks, Provectus delivered a custom machine learning solution for real-time temperature recommendations. The improved ML model enabled Sleepme to make adjustments to a user's bed temperature in minutes. Users of Sleepme’s products report a noticeable improvement in sleep quality, corroborated by data-driven sleep scores. The real-time temperature adjustment feature proved to be a significant business differentiator, enabling Sleepme to offer a higher quality of sleep to their customers and positioning them as a leader in sleep innovation.
Modern Solution to Improve Sleep Quality in Real Time
Custom ML Model Delivered in Three Weeks
Improved Sleep Scores & Customer Satisfaction
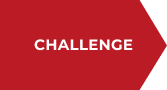
Real-Time Temperature Control as a Critical Factor Impacting Sleep Quality: Sleepme’s Vision
Sleepme is a pioneer in sleep management and monitoring technology, offering a range of products designed to enhance sleep quality. Dock Pro and Cube are smart mattress topper systems equipped with IoT-enabled sleep tracking sensors. Cube can be scheduled to cool or heat the bed using its companion application, while Dock Pro pairs with a non-wearable sleep tracker that provides real-time, AI-driven temperature adjustments.
Sleepme’s smart systems are designed to track and monitor various factors, including heart rate, respiration rate, bed pressure, and environmental conditions like temperature and humidity. By aggregating and analyzing this data, Sleepme can generate a sleep quality score, providing customers with valuable insights into their sleep patterns, to help them sleep better.
Despite their advanced technology, Sleepme was looking to further improve their line of products. The leaders of Sleepme sought to utilize AI to improve the accuracy of sleep scores by estimating the effects of changing bed temperatures. With a machine learning model at its core, this solution would enable real-time sleep score predictions by analyzing the most recent measurements during sleep. The model would also take into account various historical data and real-time inputs from IoT sensors, to achieve a higher sleep score for the night.
Sleepme wanted to gain the ability to dynamically adjust how their smart mattress topper systems cool or heat the bed, according to sensor inputs in real time. By correlating real-time environmental data with individual sleep patterns, such as restlessness and sleep cycles, they aspired to create a more adaptive sleep environment for their customers.
To accomplish their objectives, Sleepme needed a modern machine learning solution capable of processing, integrating, and analyzing various data points to deliver real-time, low-latency bed temperature recommendations. While Sleepme’s engineering team had already developed some components of the solution (i.e. a temperature optimization model), they wanted to focus on product development rather than divert resources to design, build, and handle ML infrastructure.
Sleepme was looking for a partner to help:
- Minimize infrastructure management overhead
- Establish well-defined processes
- Deliver the improved ML solution to production
Sleepme partnered with Provectus, an AWS Premier Consulting Partner with competencies in Machine Learning and Data & Analytics, to develop and deploy a robust, scalable machine learning solution, utilizing best practices for AI adoption, ML development, and MLOps. The solution would be delivered and supported through Provectus’s Managed AI Services.

Using AI/ML & IoT Data to Optimize Sleep Quality: From an ML Model to a Deployed, Real-World Solution
Provectus kicked off the project with a series of discovery sessions to examine Sleepme’s existing solution. The team assessed the company’s business requirements and expectations, established baseline metrics, identified potential risks, and operationalized the ML model.
Sleepme then completed the Proof of Concept (PoC) for their temperature optimization model. The model training and evaluation experiments were stored in Jupyter notebooks. The model was containerized using a simple Docker wrapper, and data input was implemented using JSON files.
It was clear to Provectus that the existing model needed improvements to make it fully operationalized and prepared for production use. While deploying the solution in production, best practices for MLOps were to be applied, to reduce operational overhead and complexities associated with ML development. The ML infrastructure had to be highly available and scalable, with robust monitoring and observability components.
Below is the solution’s high-level architecture, delivered and supported by Provectus:
Provectus leveraged a range of AWS services, including Amazon SageMaker suite, to expedite ML model development and deployment and establish a robust foundation for Sleepme, so they could allocate their resources to product innovation in the future.
The team set up a training pipeline, handled dataset versioning, and packaged the model on Amazon SageMaker. The service was also used for real-time model inference and monitoring. To streamline and automate the release and deployment processes, Provectus implemented CI/CD workflows. A data quality monitoring process was established to ensure the accuracy of the model’s results.
As part of Managed AI Services, Provectus offered the following:
- Managed MLOps — Provectus engineers took care of infrastructure provisioning and maintenance, access management, cost monitoring and optimization, networking, compliance support, and disaster recovery. The team also managed CI/CD configurations and ongoing maintenance.
- ML engineering — ML work prioritized model observability, maintenance, and re-training; ML pipeline maintenance, and data quality monitoring.
- Support — Provectus was responsible for troubleshooting, issue remediation, and escalation procedures.
This approach enabled Provectus to develop and deploy Sleepme’s ML solution for real-time temperature recommendations within weeks. The solution was quickly operationalized and prepared for production, all while the required improvements to effectively reduce AWS infrastructure management overhead were implemented. The Provectus team also established well-defined SLAs and processes, provided 24/7 coverage and support, and enhanced overall infrastructure stability, including production workloads and critical releases.

Better Sleep, More Life: Automating Bed Temperature Control to Maximize Sleep Quality in Real Time
With over two decades of award-winning expertise in sleep solutions, Sleepme has established itself as an authority in sleep quality. Their cooling mattress topper systems powered by AI further solidify their reputation for excellence. Provectus had the privilege of taking Sleepme’s products to the next level.
Provectus leveraged its extensive expertise in AI adoption, ML development, MLOps, and Data & Analytics to develop and deliver a modern machine learning solution, capable of providing accurate, real-time temperature recommendations and adjustments for Sleepme’s cooling mattress topper systems.
With the Provectus solution, Sleepme can now identify the optimal temperature adjustment and mirror it to a customers’s bed within minutes. For Sleepme, this feature is a key business differentiator, enabling the optimization of customers’ sleep in real time.
The machine learning model powering Sleepme’s solution was designed, built, and delivered in just three weeks. The Managed AI Services Onboarding phase spanned several months, to bring the solution to its desired state.
The ML solution was operationalized and prepared for real-world application, which included:
- Deployment of the solution and model(s) to production
- Implementation of ML & MLOps best practices
- Preparation of ML infrastructure for production use
- Adoption of data quality and data governance methods
- Utilization of best practices for monitoring and observability
With a state-of-the-art solution in place, Sleepme’s team reduced infrastructure management overhead, streamlined engineering and operational processes, and enhanced overall infrastructure stability, including production workloads and critical releases. For Sleepme, the solution freed them to focus more on product innovation, with less operational hassle.
Collaboration with Provectus was a pivotal step for Sleepme. Leveraging Provectus’ technology prowess, Sleepme was able to successfully enhance sleep quality for its customers through AI-driven temperature recommendations, and to reinforce its position as a leader in sleep innovation.
Moving Forward
- Learn more about our MLOps Platform, Managed AI Services, and ML Infrastructure
- Explore more customer success stories covering MLOps & Managed AI: Earth.com, Appen, GoCheck Kids, VTS, Lane Health
- Apply for Machine Learning Infrastructure Acceleration Program, to get started
CONTACT US
Looking to explore the solution?