Improving Customer Retention with AI-powered Churn Prediction
Audiobooks.com predicts churning customers to take preventative measures at the right time, to reduce churn through personalized product content
Audiobooks.com is a flagship audio brand of RBmedia and the world’s largest audiobook publishing company, with a catalog of more than 250,000 exclusive titles narrated by award-winning actors. The company provides digital content to libraries, schools, and retail markets.
Challenge
Audiobooks.com wanted to improve the Cost-per-Circulation (CPC) for digital content and audiobook titles on their digital platform by predicting customer churn with AI. The more circulations per customer the platform can drive, the more value it provides to their clients (authors and agents), which means a more solid bottom line.
Solution
Provectus reviewed the existing data structures to establish a foundation for machine learning pipelines, starting with churn prediction. The iterative feature engineering for churn prediction was facilitated by pipelines implemented on top of Amazon SageMaker and Kubeflow Pipelines, making it possible to improve accuracy and deliver churn estimates on new data.
Outcome
The new churn prediction model enabled Audiobooks.com to predict churning customers, reduce attrition rate, and improve CPC for selected audiobook titles. The model helped them to segment and target users more accurately, thus improving engagement, to optimize costs, drive more value for clients, and create a more robust bottom line.
Significant Reduction in Customer Churn Rate
95% Accuracy of Churn Prediction Model
POC Completed and Deployed in 5 Weeks
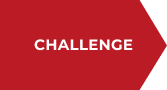
AI Helps Subscription-Driven Businesses to Prevent Churn, to Scale More Effectively
Customer retention is the primary driver of growth for subscription-based businesses like Audiobooks.com. While having the broadest distribution network, they company competes in a market where customers can easily choose from plenty of alternative providers, even within a single product category. Any bad experience can prompt a customer to abandon a brand and take their business elsewhere (i.e. churn).
Customer churn (also known as customer attrition) results in a loss of revenue, affects customer lifetime value (LTV), and makes it more expensive to acquire new customers.
For Audiobooks.com, it was critical to minimize the churn rate to lower Cost per Circulation (CPC). The metric is calculated as the total cost of their licensing fees vs. the total number of book checkouts. The more circulation per customer the platform can drive, the more value they provide to digital distributors (retailers, libraries, and school platforms), agents, and authors. A good CPC cannot be achieved with high customer churn, which negatively impacts book checkouts and makes it more difficult for distributors (mainly libraries and schools) to get government funding.
Realizing this, the executive team set a bold goal: Track the habits of problem users and proactively make personalized offers, to minimize attrition by predicting churn while increasing checkouts.
Audiobooks.com joined forces with Provectus when their marketing and AI/ML teams were already working on several initiatives to predict churn for one of their projects. The objective of Provectus was to build a more accurate and efficient churn prediction model, and to establish a robust foundation for machine learning pipelines that enable churn prediction.

Building a Churn Prediction Model Using Historical Data and Churn Events
Before moving forward with building a churn prediction model, Provectus reviewed the existing churn prediction initiatives. We set up an evaluation framework to assess data, validate and clarify hypotheses, and check churn prediction models in a consistent and reproducible manner.
The team built a dataset that consists of 2.5M user profiles and five years of historical data, such as listening activities, clicks, search and recommendation logs, subscription renewals, and churn events. Several pipelines were then developed that take this dataset and produce a deployable churn prediction model:
- Data preparation pipeline that cleans the dataset and reshapes it into a set of records feedable into a model,
- Extensible feature generation pipeline that enriches records with hundreds of feature columns that ML models operate on top of,
- Model training & evaluation pipeline that produces model artifacts and metrics,
- Model application pipeline that takes a previously trained model, applies it to new data records, and outputs model predictions.
These pipelines enabled quick experimentation, where a new feature generator was added at every successive iteration and its impact on model performance was measured. One hundred features were selected for use in a final model. Examples of those features are “Most frequent price plan,” “Days since last search,” “Number of searches,” and “Subscription credits expired last month.” When combined, these pipelines make an end-to-end pipeline that is used to make predictions with only a few clicks.
Provectus came up with a few options for how the data and machine learning infrastructure for churn prediction would look. The final variant is displayed below. It uses Kubeflow Pipelines as a pipeline orchestrator and experiment tracker, deployed on top of an Amazon EKS cluster. ETL pipeline steps are implemented as Spark applications running on Amazon EMR. Various Amazon SageMaker components are used for ML steps, e.g., Training and Batch Transform Jobs.
The churn prediction model itself was trained on historical data. The final model’s precision and recall did not drop below 95% in different testing scenarios, with its Decision Tree, Random Forest, and CatBoost variants showing 98-99% accuracy. Along the way, the team detected and solved several bottlenecks, and developed required pipelines to enable Audiobooks.com to retrain the model and to improve churn prediction accuracy on real-world data as the project develops.
Given the nature of the task, it was important for Audiobooks.com to have the means to explain churn prediction results. The pipeline built by Provectus allowed the team to see which features influenced churn prediction the most, to come up with more effective strategies to prevent attrition.

Churn Predictions Reduce Customer Acquisition & Retention Costs, and Increase Growth Potential
In a subscription-based business model, high churn rate is the strongest predictor of slow growth. High attrition companies invest massive resources to acquire new customers and retain existing ones, which stifles their potential to scale their business. The team at Audiobooks.com did not want to follow that path.
The churn prediction model, built by Provectus in just five weeks, allowed them to look into and analyze the specific reasons customers were about to churn. This helped them devise strategies to efficiently counter attrition through personalized offerings, bonus programs, and other incentives.
Because Provectus delivered a fully scalable model and the required toolsets, Audiobooks.com was able to run and modify the model(s) on their own, by manually combining different features and adding hidden patterns into the mix. They used their data tools for data processing and analytics as well.
The model’s output streamlined manual marketing effort to reduce churn, making it possible for them not to spam users while also delivering the correct messaging at the right time. With that in mind, the team was looking to take automation to the next level and build their messaging around the outcomes and feedback into the model for full automation of churn reduction.
With the help of its new, highly accurate churn prediction model, Audiobooks.com managed to significantly reduce their monthly churn rate. The reduction in churn had an almost immediate impact on Cost per Circulation, book checkouts, and LTV, all of which strengthened their position in the digital content market.
Moving Forward
- Learn more about the Provectus Customer Retention Optimization (Customer 360) solution
- Read the article on AI use case prioritization for your organization
- Apply for ML Use Case Discovery Program to get started
CONTACT US!
Looking to explore the solution?