The CxO Guide to Generative AI:
Threats and Opportunities
Yes, It’s real!
Over the last few months, we’ve had conversations with leadership teams that start with a high-level question: “Will Generative AI impact our business, and how? The board wants us to develop a Generative AI strategy.”
With a certain level of confidence the answer is YES, Generative AI will impact all industries, directly or indirectly.
According to OpenAI Research, around 80% of the U.S. workforce could have at least 10% of their work tasks affected by the introduction of Large Language Models like ChatGPT, while approximately 19% of workers may see at least 50% of their tasks impacted.
As of June 2023, the majority of CEOs and business owners have already experienced the impact of ChatGPT in their personal lives, noticed its effects on professional productivity, and discussed it in strategic business conversations.
This report is focused on practical opportunities, threats, and limitations of the state-of-the-art Generative AI applications for enterprises of all sizes. We’ll review business patterns, as well as technical, legal, security, and governance aspects to consider when transforming the enterprise with Generative AI.
If you are a shareholder, board member or CEO, it is imperative to develop a Generative AI strategy.
Background and baseline alignment: What is actually happening and why?
AI, Generative AI, GPT, Large Language Models, and Foundation Models
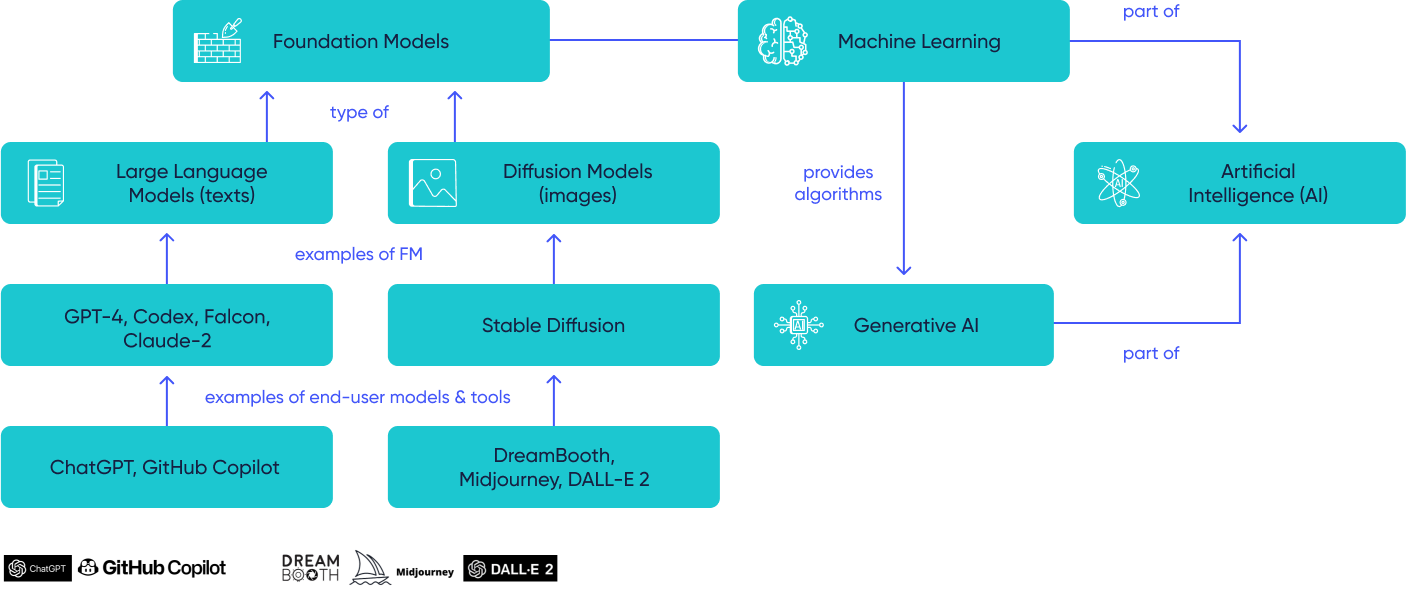
Existing applications in everyday life that you may never have noticed
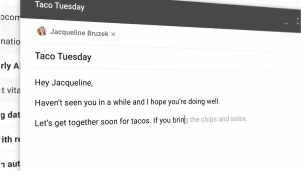
LLMs help to overcome writer’s block in Gmail or Outlook
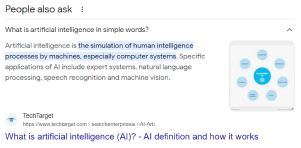
Google Search uses LLM models to summarize answers that appear on the first page
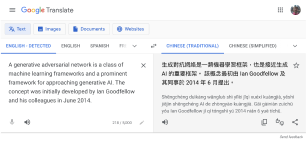
Translation and speech recognition services are the first widely adopted applications of transformer models
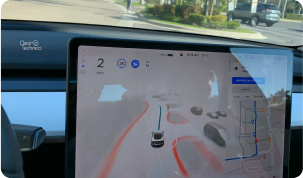
While driving, the Tesla’s autopilot is powered by the similar architecture of pre-trained transformers
“Stop AI for 6 months.” Elon Musk, Bill Gates, and 1000+ others signed a petition to halt AI experiments.
Are humans going to be replaced by AI in the future?
CEOs might not be aware of all technical details to innovate on business models with Generative AI.
GPT applications are not secure and not compliant, so major enterprises and even whole countries ban it.
- Open-source software can’t be regulated or stopped at its core.
- There are a lot of harmful use cases like generation of new chemical weapons. Appropriate programs to address the issue must be developed by regulators in collaboration with major AI players.
No. Generative AI is a powerful yet practical technology that has its own inputs, outputs, and limitations. We suggest not to extrapolate it to general intelligence, and to completely eliminate this distractor from real-world business conversations.
Yes and No. “We too” low-hanging-fruit use cases are going to be commoditized soon. Really innovative business models, however, require a much deeper understanding of both LLMs and first principles of Generative AI.
No. It is recommended not to confuse Generative AI technology with applications like ChatGPT that are not designed for enterprise requirements. But there are multiple vendors, including major cloud providers, who address or will address these issues soon.
It is important to align on frequently asked questions and common distractors. Doing so will help businesses leaders eliminate noise and stay focused on achieving real business outcomes.
Why the market shift is happening right now
It didn't happen overnight. Two major forces have converged and pushed
the technological breakthrough that we are all experiencing today:
1
Advancements in deep learning, generative research, foundation models over the last 15 years paved the path for existing state-of-the-art model architectures. “Attention is all you need” – a breakthrough paper from 2017 is considered as a starting point of the most recent innovation cycle. It took the industry 5 years to build an actual user facing application (ChatGPT) and develop a commercial model for selling it to end users.
2
Data storage, Cloud & GPU compute became more accessible. Prior advantages of internet giants and their almost exclusive access to unlimited scale is no longer the case. Small startups like OpenAI and Stability AI can now develop new breakthrough applications on the same infrastructure as Google or Meta.
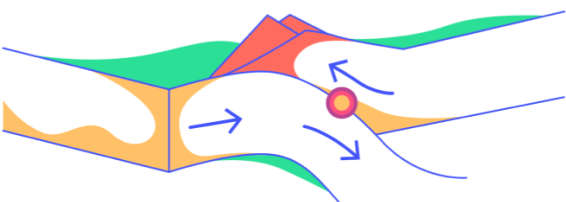
Don’t wait until your industry is disrupted by AI



The strategic question that must be raised on every board agenda:
“Will our industry and business model be relevant in 10 years?”
“If AI can drive a car, I’m sure it will disrupt my industry sooner or later.”
– Brij Patel, Founder and CEO of AFG
An example of an immediate protective legal action item for content-heavy businesses is to update their data terms of use.
Discover your competitive
advantage: Emerging
opportunities and use cases
Assess two major types of opportunities
Examples:
Tesla is monetizing its enhanced autopilot and self-driving add-ons for $6k and $15k each, respectively, which account for 50% of the total car price. In this sense, Tesla is becoming an AI business.
GitHub is charging $19 per enterprise user for its AI Copilot add-on, representing a 100% price increase from the basic plan.
Bloomberg has released BloombergGPT, creating an opportunity to begin monetizing its data assets through a new interface.
Examples:
Generative AI boosts worker productivity by 14% in the first real-world study.
At Provectus, we see a productivity increase of 50-90% in tasks such as new domain research and business analysis. We’ve also seen a 40-80% productivity boost in the generation of synthetic datasets for ML model training and quality assurance. Engineering tasks – from requirement analysis and legacy code base research to bootstrapping new applications, testing, and debugging – have seen a performance improvement of 30-60%.
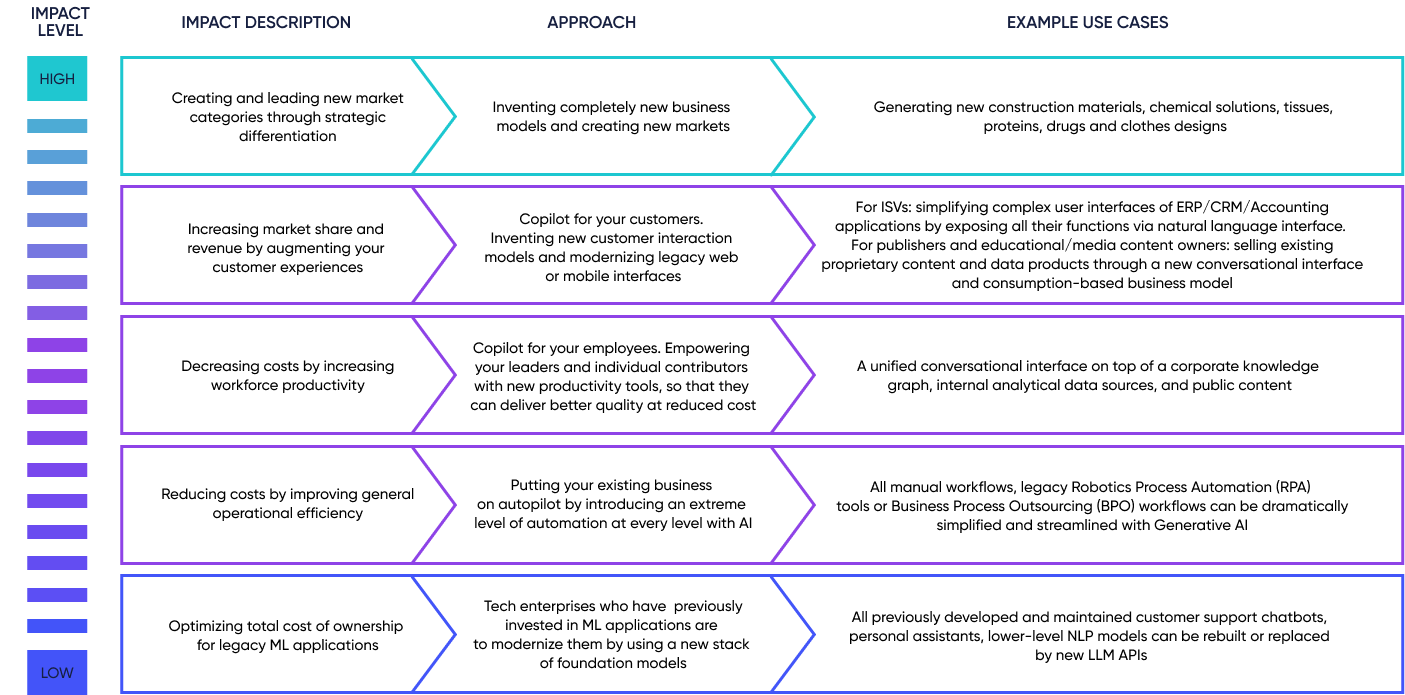
Novel materials generation
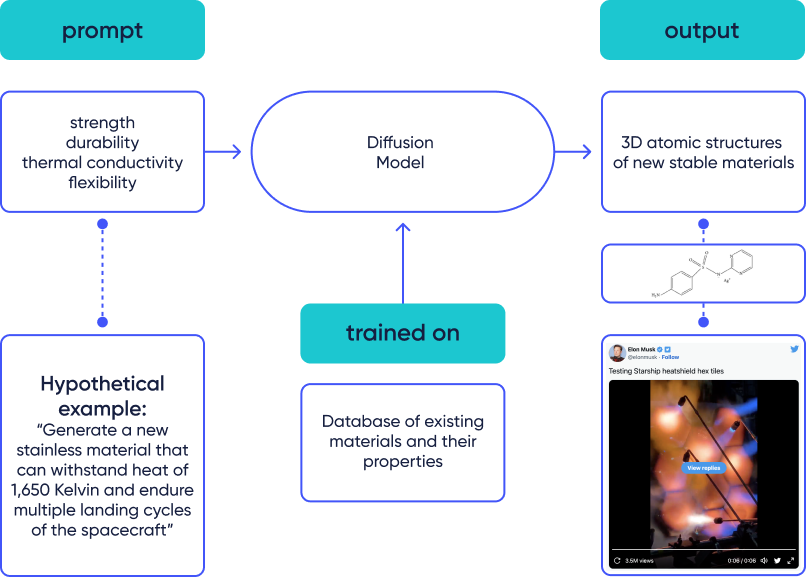

- Content search and discovery
- Content generation (text, art, music)
- Content moderation
- Scenario development
- NPC generation in gaming
- Commentary generation for sports events

- Horizontal and vertical search transformation and market value redistribution
- Augmented customer experience
- Specialized personal AI agents
- Content licensing for AI vendors

- Specialized productivity tools
- Internal knowledge graphs
- Conversational business intelligence
- Client reporting generation

- Client reporting and compliance reporting
- Credit spreading
- Intelligent document processing and understanding
- Intelligent due diligence
- Augmented client interactions

- Micro learning and AI-augmented experience
- Educational content monetization through conversational UX
- Educational and training content generation and new monetization models

- Medical charts and clinical notes generation and understanding
- Pricing and reimbursement modeling
- R&D knowledge graphs
- Protein folding simulation
- Creation of new drugs, proteins and materials, and their assessment
Client reporting in Financial Services
- Time to produce and deliver a report to the client, leading to increased customer satisfaction
- Cost of producing and maintaining client and compliance reporting, directly impacting the operating margin
- Fiduciary duty for providing accurate and valid information
- Data lineage and cross validation of every metric and data point in the report.

- Conversational business intelligence and solutions for tapping into company’s data and insights
- Augmented communication strategy:
- Board reporting
- All-hands updates
- Company values

- Sales call assistant
- Market research and reporting
- Proposal generation
- Prospect research and due diligence

- Improving employee experience through internal knowledge graph and AI search
- Augmented onboarding
- Augmented screening interview

- Industry specific R&D knowledge graphs and AI-curated industry insights
- Simulation and experiments tracking
- New materials, chemicals, protein designs

- Compliance reporting
- Security risk assessment
- Augmented security training
- Background check

- Augmented contract review and redlining
- Vendor assessment and due diligence
- Reporting
- Scenario simulation and stress testing
Supply Chain Visibility & Intelligence
- Revenue through proactive mitigation of supply chain disruptions
- Operational efficiency of the command center
- Overall maturity of internal IT systems
- Adoption and change management of legacy business processes
How to start
a Generative AI journey?
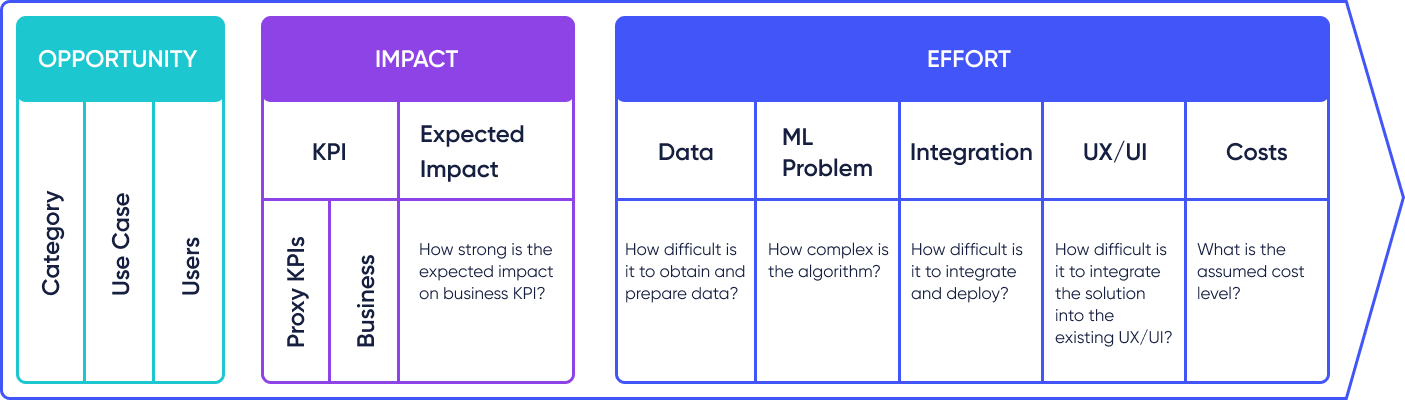
You need to have a hands-on AI Expert and AI Product Owner in the room for a quick feasibility assessment, and to create a high-level ROI model
No matter how excited you are about technology, try to find a solution for your business problem, and not vice versa
Challenge your use cases for compliance and fairness requirements early on in the process
Opportunity-Risk-Strategy Tool
Use Case 1:
Customer support (business function)
High impact on customer satisfaction
Low risk perceived – these jobs are already commoditized and outsourced
Executive consideration
Introduce the Generative AI-powered chatbot as an entry point for customer inquiries to make human agents assist only with unresolved cases
Where to start
Focus on business outcomes – test drive a solution pilot with a small subset of the audience, fine-tune, and scale.
Use case 2:
Content creation (individual contributors)
High impact on productivity
High risk perceived – a perceived threat of workforce elimination
Executive consideration
Redefine the role of content creators to shift their focus on providing inputs and supervising the AI-generated content vs. creating the first drafts
Where to start
Focus on change management – cultivate an AI-first culture and build from success with early adopters within the team.
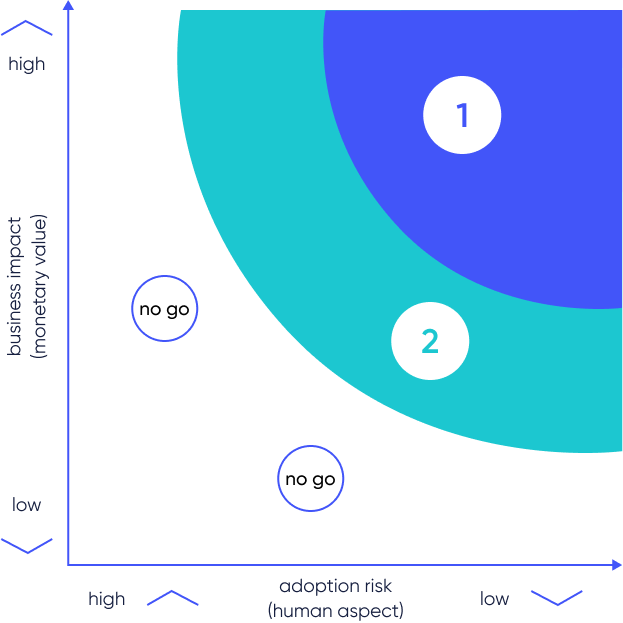
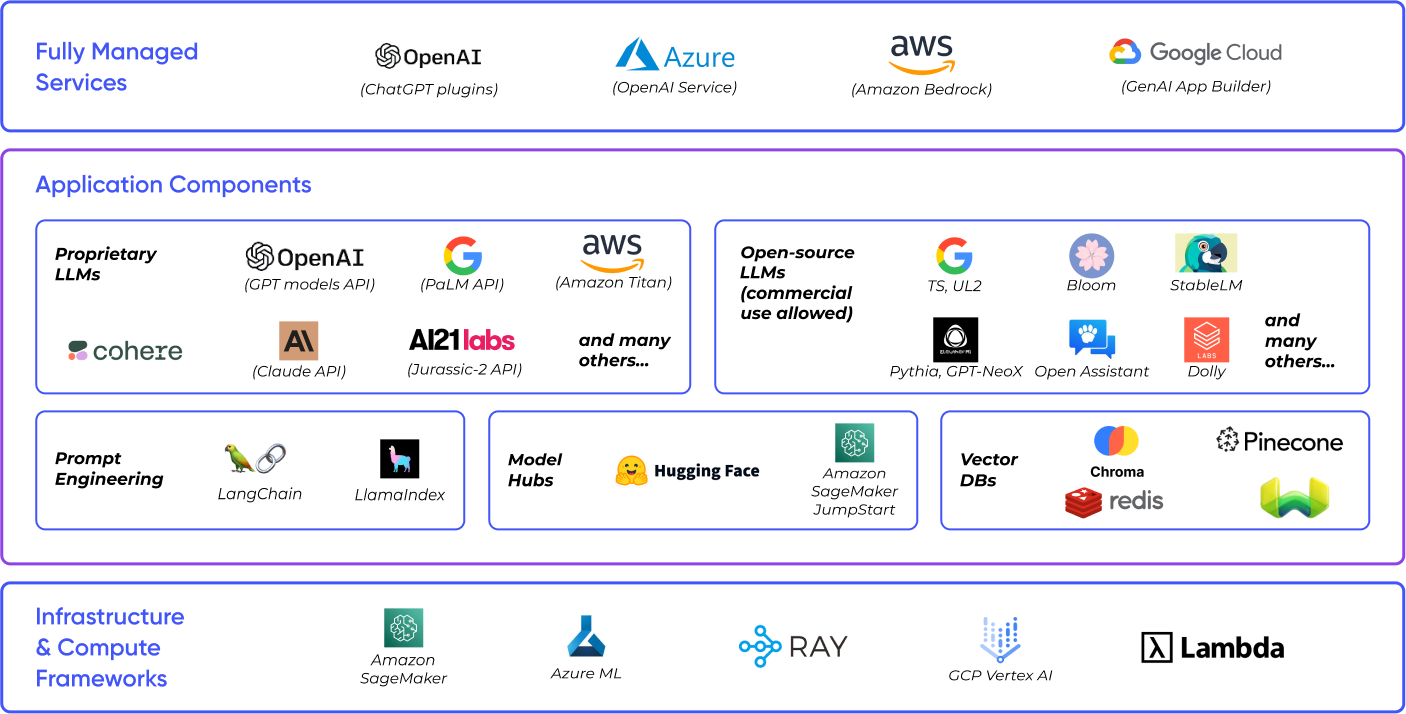
- Design human-in-the-loop experience for continuous evaluation and data collection. Don’t use LLM as part of a fully automated pipeline without human in the loop
- User training and clear communication about possible AI behavior
- Apply LLM alignment methods like Reinforcement Learning from Human Feedback (RLHF). Note: it is an advanced technique mastered by a handful of LM vendors so far.
- Turn hallucinations into a positive benefit for creative use cases like music and art generation.
- Budget carbon impact in advance when selecting an LLM vendor and include it into the total cost of the model
- Stanford AI Index report is one of the latest data sources for estimating an environmental impact of LLMs
- Multiple deterministic systems (databases & APIs) can be integrated together and be exposed through an AI interface
- Private VPC deployment of your own model, as well as 3rd-party models, is available for private deployment
- Implement logging and audit trail for capturing, filtering, and blocking of any sensitive requests to AI models
- Integration with real-time data sources and APIs, and with internal knowledge graphs
- Implementing data lineage, data cleansing, and data testing best practices
- New legal frameworks and processes to be implemented
- Mix large & expensive models for creative tasks and your own “smaller” models for predictive tasks
- Right now it’s a dawn for open-source LLMs, datasets, and optimization techniques
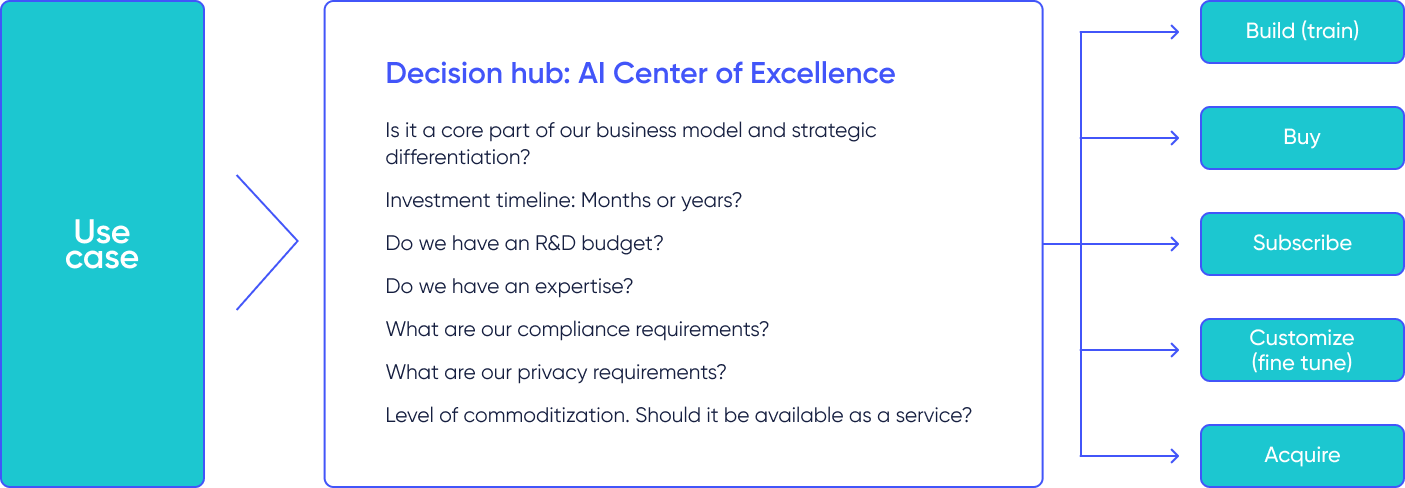