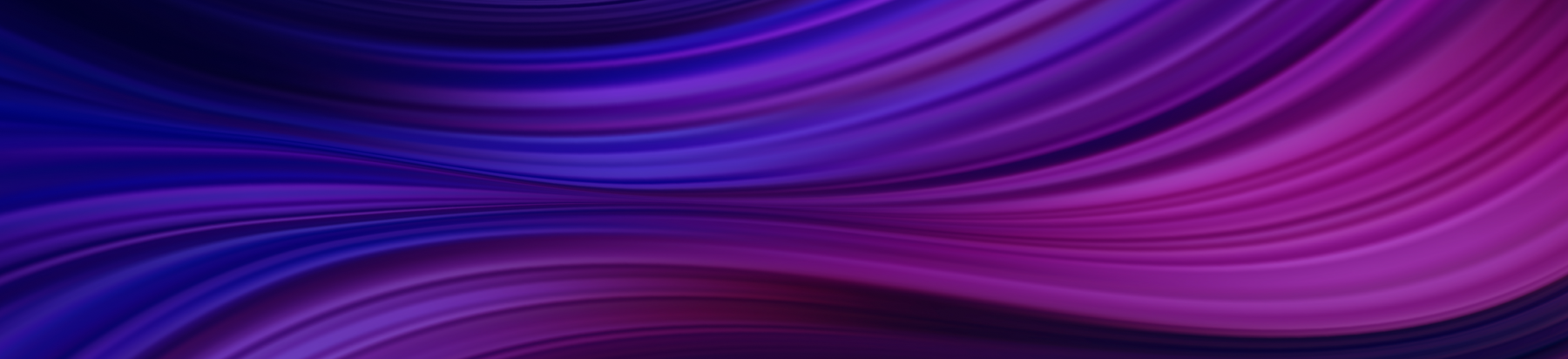
February 2, 2023
7 min read
Customer-Centric AI Transformation: Common Mistakes and Best Practices
Author:
Stepan Pushkarev, CTO and Co-Founder at Provectus
Enterprises of any size, level of maturity, industry or geographic location are constantly planning and executing new innovations: from executing digital transformations and cloud migrations to leveraging the most recent AI transformations. These waves of modernization are interconnected and often overlap each other, but the end goal of most successful CEOs is:
To provide better and more personalized customer experience.
Bear in mind that customer experience is not just about introducing personalized product recommendations for eCommerce websites. For instance:
- When CPG enterprises optimize their supply chain or last mile delivery logistics, it leads to better customer experience because customers get their products faster.
- When pharmaceutical companies migrate their legacy applications and data warehouses to the cloud, the long term goal is not only to reduce data center costs, but also to accelerate the drug discovery process. By using novel deep learning models, they are able to deliver new and personalized drugs to patients faster and more reliably.
- When manufacturing companies introduce new computer vision-based defect detection systems, it not only saves money, but also and more importantly provides quality products to their customers.
Customer 360, or Customer-Centric AI, describes a meta use case that embraces all possible use cases and endpoint solutions, to provide better and more personalized customer experience, both directly, and across all channels and touchpoints.
While the connection between AI transformation and customer satisfaction may seem obvious to some, many companies struggle with the why question. They often lack a clear vision, only making transformations because their competitors are doing so, or because an analyst advised them to.
This leads to issues with use case prioritization and skewed use case objectives. For example:
- A social network may start recommending viral and fabricated content that may improve some product metrics, but in the long run it damages customer experience.
- Or consider call center and customer support use cases: if a North Star metric is only cost reduction as defined by many CIOs, introducing AI assistants will never lead to better customer experience.
The main factors supporting Customer Centric AI Transformation are:
- The majority of enterprises have become customer-focused like never before. CPG brands, manufacturers, airlines, pharma and many other industries are now selling directly to consumers, either through marketplaces like Amazon, or directly through stores like Nike or Apple, or by building their own airline terminals and managing the customer journey from end-to-end.
- In the post-Covid world, businesses need to completely reimagine customer experience. Omni-channel is not enough. They need to go back to the drawing board and rebuild their entire business models in customer-centric ways.
Six Common Mistakes and Best Practices for AI Transformation
Challenge: Excessively massive and never-ending modernization programs
Many CIOs execute the transformation journey in a specific sequence. They begin by digitizing company assets and business processes, then hire expensive consultants to guide them through an agile transformation. Next, workloads are migrated to the cloud and a data warehouse is built so that data can be used for analytics and decision-making. Only then do they start thinking about customer-centric use cases. Building such massive programs can take five to 10 years, and they often fail badly.
Solution: Begin with customer-centric AI as the end goal
A better approach is to reimagine your customer experience and business model, then work backwards, focusing on only the most necessary components. Ninety percent of legacy applications and databases are not necessary to enable your new business. Even if your data is locked in the mainframes or in Oracle, it is possible to stream only the necessary tables to the cloud and build a new cloud native product recommendation service in just weeks. By doing so, you organically create a new foundation and best practices for the next generation of use cases, and eventually for the entire infrastructure. There is no reason to spend years moving accounting software to the cloud, decomposing your monolith into microservices, or building a data lake just for the sake of having one, before initiating AI use cases.
Challenge: Managing business expectations for AI
Most of us have an intuitive understanding of how Machine Learning and Artificial Intelligence work, but when it comes to budgeting, product, and project management of AI use cases, and integrating them into business processes, business stakeholders and senior management may not fully understand the various upstream and downstream implications.
Solution: Management bootcamp
One full week of management training is enough to get your managers and stakeholders up to speed. At Provectus, we provide detailed blueprints for use case prioritization and definition, use case feasibility studies, budgeting, project management, quality assurance and an overall understanding of how machine learning works. Unlike traditional IT projects, AI projects may require budgeting for quality dataset or GPU infrastructure. Also, AI project management cannot be tied to scrum sprints with predictable outcomes. They must be based on iterations around ML experiments and metrics improvements.
Challenge: Organizational silos and culture
Many enterprises have functional service teams (like UI, platform, data engineering, DevOps, etc.) and business units. AI projects, especially in the beginning phases, require the full collaboration of all functional teams and business units. The PMO office often enters the picture as well, to try to manage it all. This approach is likely to fail.
Solution: A cross-functional AI team
AI requires a much more diverse and cross-functional team compared to traditional IT projects. Take a top-down approach by first assembling your AI team, giving them the autonomy to execute the project with the support of others. A recommended team composition to start with could be: Domain expert & Business Leader, AI/ML Solutions Architect, ML Engineer, Data Engineer, Infrastructure and Security Specialist, UI Designer, Software Engineer, Data and ML QA Specialist.
Challenge: IT and security
Enterprises have been protecting IT infrastructure and data from their engineers and employees for decades. Machine Learning disrupts this legacy foundation by asking for data. When not aligned properly, IT becomes a major bottleneck to data acquisition.
Solution: Include IT in your game plan
IT should not be treated as an external enemy. Your IT and Security Specialist should be involved as early as possible and given a sense of ownership in executing an AI use case. Modern Data and ML Infrastructure solutions handle security very seriously, and most cloud providers and professional service firms that specialize in MLOps can provide data and ML engineers with well-architected blueprints for secure and reliable data access.
Challenge: Unclear business outcomes
It is impossible to change a single feature in a product to make it great again. In the same way, ML metrics cannot be strongly connected with business KPIs. For example, the hypothesis that targeted email campaigns will improve click rates and lead to more product purchases can be proven right or wrong. Netflix has hundreds of ML models to power user experience, and solving a single bottleneck will not necessarily lead to tangible improvements.
Solution: Time to scale
Scaling should be at least partially covered during Management Bootcamp, and also thoughtfully managed during execution, and while setting expectations for each use case. Very often, the first baseline model has a very clear learning curve that can take up to 3-6 months of training/retraining on new production datasets before demonstrating desirable performance. All these aspects, including time to value, must be considered when prioritizing use cases, and the role of the AI/ML Solutions Architect is essential in this process.
Challenge: Inability to scale beyond one or two use cases
Scaling one AI use case to production size is hard, but it is even more challenging to design and build an ecosystem that can serve as a robust foundation for a scalable AI growth across the organization. This sophisticated, end-to-end ecosystem should encompass multiple data, technology (infrastructure), and business components (e.g. explainability UI) to help generate value, not cause problems. Developing such an ecosystem takes a lot of time, money, talent, and unwavering commitment from leadership.
Solution: Establish a central AI Hub
Midterm solutions require the building of a central and very agile AI Hub that helps to build reusable components for fast tracking multiple use cases in parallel: reusable datasets, pipelines, features, notebooks, ML models. A long term strategy requires a fundamental organizational shift from business-driven to mission-driven, to give people on all levels enough context. They should be able to innovate quickly and independently, bringing new use cases that contribute to the single vision of a Customer Centric enterprise.
Summary and Steps Moving Forward
- Define vision and strategy for the next innovation cycle with AI. Customer Centric AI is not for everything and everybody, but it creates good alignment, increases the probability of success, and provides immediate benefits to the business.
- Prioritize 3-5 most impactful Customer Centric use cases and start working backwards, without losing focus or spending time on science experiments and extensive all-inclusive modernization programs. Be ready to fail fast with one use case, but successfully deploy others.
Break down organizational walls and build an autonomous high-performing AI team that is aligned with the vision. - Scale success by adding more teams and reusing foundational components. Set a long term goal to flip the top-down process and empower AI innovation from the bottom up.