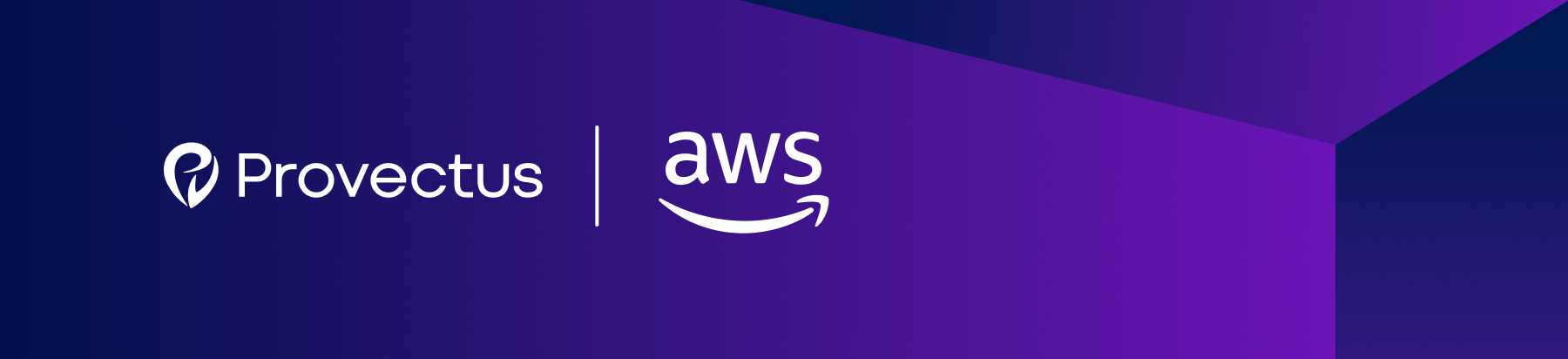
July 13, 2023
1 min read
How Provectus helped Earth.com to Implement MLOps Infrastructure for Image Recognition App
Authors:
Marat Adayev, Machine Learning Solutions Architect – Provectus
Dmitrii Evstiukhin, Director of Managed Services — Provectus
James Burdon, Senior Solutions Architect — Amazon Web Services
The deployment of machine learning (ML) models into production environments can present a challenge, particularly when the models are already driving significant real-world business decisions. Machine Learning Operations (MLOps) – the practice of reliably and efficiently deploying and maintaining machine learning models – provides a technical response to this challenge.
At-scale real-time image recognition is one of the technical problems that requires the implementation of MLOps to account for various alterations in data, models, and concepts.
EarthSnap is an AI-powered nature identification application developed by Earth.com. EarthSnap harnesses the power of AI/ML to enable users to quickly and easily identify various plant and animal species via their smartphone cameras, in real time.
This article dissects the journey leading to the release of the AI-powered EarthSnap application, illustrating the pivotal role of MLOps and ML infrastructure in the development, deployment, and ongoing management of its ML models.
Provectus, an AWS Premier Consulting Partner, has carved its niche in Machine Learning, Data & Analytics, and DevOps. Provectus was instrumental in augmenting EarthSnap’s AI-powered image recognition capabilities, helping Earth.com to reduce engineering heavy lifting and minimize administrative costs. The team accomplished this by implementing end-to-end ML pipelines that were seamlessly integrated as part of a managed MLOps platform, and further supported by a suite of managed AI services.
Read this article on the AWS blog to learn more about the Provectus approach to developing and implementing MLOps infrastructure for Earth.com.