Empowering Caregivers with Accurate AI Predictions
Gale accurately predicts shift fill rates for nurses and caregivers with AI/ML, to improve staffing predictability, increase operational efficiency, and improve care delivery
Gale Healthcare Solutions leverages digital technology to connect healthcare facilities with a network of over 64,300 credentialed and qualified nurses and caregivers. Their services, which include recruiting, scheduling, and payroll management, ensure that local healthcare facilities have access to competent professionals to meet their needs. The Gale app provides flexible schedules and daily pay for nurses, and consistent healthcare worker shift coverage for facilities, which results in quality care for patients.
Challenge
Gale sought a modern solution to optimize their technology-driven healthcare marketplace. The envisioned ML solution would predict shift fill rates, to help improve shift booking and predictability. It would analyze patterns in missed nurse shifts and highlight to management which shifts are most likely to need attention. The goal was to reduce the number of mismanaged shifts, improve patient care, and increase operational efficiency.
Solution
Provectus developed the ML solution for predicting shift fill rates on AWS. It enabled Gale to predict whether a shift was likely to be filled or not, giving them a powerful tool to optimize staffing and operate with greater precision. The solution also helped Gale decrease the number of excess notifications to their users, so that messages sent from Gale to their nurses and caregivers result in open shifts being filled more often.
Outcome
Provectus worked with Gale to design and build a shift fill rate prediction solution. By rapidly testing assumptions and iterating where improvements could be made, Provectus delivered the ML solution within six weeks. By adopting AI/ML, Gale was able to optimize healthcare staffing, improve care delivery, and strengthen its bottom line. Gale now considers Provectus as an AI/ML partner to accelerate their journey towards data-driven enterprise.
7%
Improvement in Shift Fill Rate
75%
Accuracy of the delivered ML model
6 Weeks
From ML project inception to delivery
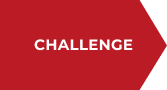
Accurate Shift Fill Rate Prediction as a Foundation for Optimized Staffing and Quality Care
Gale Healthcare Solutions is a company that leverages digital technology to connect healthcare facilities with qualified nurses and caregivers. They have a strong network of over 64,300 healthcare workers, which is growing daily.
Gale’s primary services include:
- Recruiting: With over 30 years of healthcare staffing experience, Gale specializes in finding and verifying the credentials of qualified nurses to meet the needs of local healthcare facilities.
- Scheduling: Gale uses on-demand technology to simplify scheduling by connecting available nurses to open shifts at the touch of a button.
- Payroll: Gale offers nurses the option to be paid daily within a few hours of completing their shift.
Gale’s platform, the Gale App, is designed to connect nurses and caregivers to healthcare facilities in need of clinical support. The app provides flexible schedules and daily pay for nurses, consistent coverage for facilities, and quality care for patients.
As a healthcare marketplace, Gale relies on the gross margin between rates paid by healthcare facilities and nurses registered in the system. This necessitates that Gale leaders prioritize three major business goals:
- Optimizing staffing through improved scheduling
- Ensuring the highest quality of healthcare is provided
- Delivering a user-friendly experience to nurses and healthcare facilities
To meet these goals, Gale leaders were looking to develop a machine-learning solution that would help their operations managers to search for shifts according to their likelihood of being filled, and zero in on those less likely to be filled. The solution would also help Gale decrease the number of excess notifications to users in the system, resulting in open shifts being filled more quickly and accurately.
The adoption of the ML solution for shift fill rate prediction would reinvent Gale’s operations in two significant ways:
- Accurate prediction of shift fill rates leads to improved scheduling and reduced burnout for nurses. It ensures that there are enough nurses on duty for each shift, reducing the likelihood of last-minute schedule changes, thus improving job satisfaction among nurses. This allows to optimize staffing, thus strengthening Gale’s bottom line.
- The ML solution impacts the quality of care provided to patients. If the model inaccurately predicts staffing needs, it could lead to understaffing or overstaffing. Understaffing can result in nurses at local healthcare facilities being overworked, leading to decreased quality of provided care, while overstaffing can lead to unnecessary costs to Gale.
Because staffing services form the core of Gale’s business, it was crucial to optimize the logistics of scheduling and ensure proper shift coverage. This would improve the company’s revenue and provide Gale with opportunities for further market expansion.
Gale’s data science team was tasked to build the envisioned machine-learning solution for shift fill rate prediction. However, it quickly became evident that they needed support from a third-party company with strong experience in ML Engineering and Data Analytics on Amazon Web Services (AWS).
Provectus, an AWS Premier Consulting Partner with competencies in Machine Learning and Data & Analytics, was selected to join the Gale project through the AWS Fast Start Program. Provectus would develop a highly accurate ML model, with all required Data/ML infrastructure components, to predict how likely open shifts were to be filled, to help Gale decrease the number of missed shifts, optimize staffing and operations, and increase overall revenue.

From Data Analysis to Shift Fill Rate Predictions: Provectus’ Solution for Gale
Provectus examined Gale’s business model and use cases, while also conducting an in-depth analysis of all available data. After a thorough review and discussion of all specifics related to the selected use case (i.e. ML model for shift fill rate prediction), the team began work on a Proof of Concept (PoC).
Provectus designed and built a comprehensive shift fill rate prediction solution, powered by machine learning, in three main stages:
#1 Exploratory Data Analysis and Modeling
In the initial phase, Provectus defined data schemas, including raw data, model input data, and pipeline inputs and outputs. The team provided suggestions for cleaning data and identified data gaps that could impact model accuracy. Various hypotheses for feature engineering and baseline implementation were explored and evaluated, laying the groundwork for the baseline classification model. A robust model evaluation framework was established to assess the model’s performance and ensure that it met standards for accuracy and reliability.
#2 Training Pipeline Development
The training pipeline was the next critical phase. It began with data ingestion in training mode, followed by data preprocessing. The model’s hyperparameters were fine-tuned to optimize its performance. After the model was trained, it underwent a thorough evaluation to ensure that it was ready for deployment.
#3 Batch-mode Inference Pipeline Implementation
The final stage was the batch-mode inference pipeline. This involved data ingestion in inference mode and data preprocessing. The batch transformation process was then carried out, followed by the export of predictions. The pipeline allowed the model to analyze and understand shift fill patterns on a larger scale, providing Gale with actionable insights to optimize their staffing services.
Following is the architecture diagram of the delivered ML solution for shift fill rate prediction:
Within a span of six weeks, Provectus successfully performed EDA, implemented and evaluated the baseline model, and achieved the desired model metrics with 75% accuracy and 85% recall. In addition, the entire machine learning workflow was transitioned to Amazon SageMaker Pipelines.
The PoC was implemented in Amazon SageMaker Notebooks, utilizing the CatBoost library for model building. Amazon SageMaker Pipelines service was employed to automate the machine learning workflow, ensuring a seamless and efficient ML development and deployment process.
As a result, Gale received a fully functional ML solution, ready for integration with their Shift Management Platform. The model predicts the Shift Fill Index (SFI), providing guidance to personnel on which shifts are less likely to be filled, and may require manual intervention or push notifications to caregivers.

Optimizing Shift Management in Healthcare: Increased Shift Fill Rates, Improved Booking and Predictability
The collaboration between Gale Healthcare Solutions and Provectus led to significant improvements in operational efficiency and revenue growth. The ML solution streamlined the shift management process, enabling Gale to:
- Increase shift fill rates
- Improve booking and staffing predictability
- Enhance communication between healthcare facilities and nurses
The shift fill rate prediction solution developed by Provectus resulted in a 7% increase in shift fill rate since its launch. This predictive model allowed Gale to focus their efforts on shifts that required more intervention, reducing understaffing or overstaffing, and improving patient care.
The Provectus solution enabled Gale to streamline the communication process between nurses and healthcare facilities, reducing the number of excess notifications and making each interaction more meaningful. This led to open shifts being filled faster and more often, further optimizing Gale’s operations and revenue.
The success of this ML project has positioned Provectus as a key partner in Gale’s growth strategy. The rapid testing and iteration approach adopted by Provectus not only helped develop and deliver the ML model quickly, but also demonstrated to Gale stakeholders the business impact of AI/ML in weeks.
Provectus has outlined next steps in Gale’s machine learning roadmap, which include: CI/CD; automation of model deployment, retraining and monitoring; and the integration of a feature store. With the help of Provectus, Gale is now ready for their next step in a full-scale AI transformation on AWS.
Moving Forward
- Learn more about the Provectus ML Infrastructure
- Explore more customer success stories covering ML use cases: Forge, Carson, GoCheck Kids, VTS
- Apply for Machine Learning Infrastructure Acceleration Program, to get started
CONTACT US
Looking to explore the solution?