Transforming Risk Management in Insurance Underwriting with Generative AI
Convex speeds up and scales underwriters’ decision-making by using AI to make sense of risk reports
Convex is an international specialty insurance company with operations in Bermuda, Europe, the UK, and the US, with over $3B in long-term capital raised. It combines its unparalleled experience and reputation with a legacy-free balance sheet, offering clients a wide range of insurance and reinsurance products, and digital risk management solutions. Convex's financial strength is underscored by its excellent ratings from AM Best and Standard & Poors.
Challenge
Convex saw an opportunity to reinvent its underwriting process by using generative AI, to help underwriters with decision-making. For some lines of business, they were struggling to distill hundreds of pages of risk reports within the limited timeframe given by brokers. By integrating GenAI capabilities, Convex hoped to accelerate decision-making while maintaining high standards of accuracy and judgment crucial in the insurance industry.
Solution
Provectus developed a state-of-the-art GenAI solution that automatically summarizes risk reports, extracting key details of interest, their sentiments, and metrics. At the core of the solution is Cohere Command, an LLM hosted on Amazon Bedrock. Provectus applied the in-context learning approach to perform highly accurate summarization and extraction tasks, specifically tailored to risk reports in PDF format.
Outcome
Convex received a modern solution that can process and analyze risk reports, and generate summaries that can be either viewed on the web or exported as a
PDF. These summaries replicate the elements that its SMEs produce manually, helping underwriters to assess risks and make decisions faster, at scale. The summarization process takes mere minutes per report, enabling Convex to process more risk per underwriter.
3 weeks
From the project’s inception to a Prototype in production
10 min.
From a 100-page report to a comprehensive summary
100x
More risk reports analyzed leading to better decisions and insights
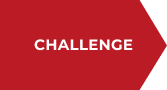
Streamlining Risk Assessment in Insurance Underwriting Through AI & Automation
Convex’ leaders are committed to reinventing the business model through end-to-end digitalization of operations, and by empowering data-driven decision-making for all roles and functions. With new advancements in generative AI and Large Language Models (LLMs), they saw an opportunity to enhance efficiencies throughout the organization.
Insurance underwriting is one area that was strategically prioritized to be simplified by technology such as generative AI. By leveraging generative AI, the leadership expected their underwriters to be able to assess risks more quickly and to improve client service. They would no longer need to wait for subject matter experts (SMEs) to summarize complex risk reports.
Previously, before the underwriters could make a decision on a risk, subject matter experts had to manually evaluate 100-page risk reports that included all technical details of the object. The SMEs would spend hours objectively reviewing and summarizing these reports for the underwriter.
Convex joined forces with Provectus, an AWS Premier Consulting Partner, to develop and deploy a state-of-the-art report summarization and information extraction solution powered by generative AI and Cohere’s Large Language Models, hosted on AWS.

Going Beyond Manual Report Summarization with GenAI and Cohere’s LLMs on AWS
Provectus tackled the project in two major phases:
- The first phase centered on developing a solution prototype. The Provectus team quickly adapted Cohere’s Command Large Language Model (LLM), hosted on Amazon Bedrock, to summarize and extract engineering reports in PDF format. The team collaborated with SMEs to incorporate feedback.
- The second phase focused on operationalizing and deploying the GenAI solution for real-world use. This included setting up end-to-end ML pipelines, establishing CI/CD processes, implementing model versioning and monitoring, and delivering a user-friendly UI/UX for their underwriters.
Let’s examine these two phases more closely.
Phase 1: Prototype Development
Provectus designed and built a prototype of the solution for processing of lengthy engineering reports. The solution would extract key metrics from the report, classify and summarise the text while indicating sentiments.
While the process described can be decomposed in a few tasks from the Natural Language Processing (NLP) realm, it was clear that Convex had to leverage generative AI and Large Language Models. This was due to the complexity of the language and domain in the input reports, the volume and size of the reports, the scattered nature of the information to be distilled, and the limited availability of labeled data.
Below is the high-level architecture diagram of the solution proposed by Provectus.
Provectus rapidly developed the proposed solution using AWS services, third-party platforms, and open-source frameworks. Central to this solution is Cohere’s Command LLM, accessible via Amazon Bedrock.
The Provectus ML team established a comprehensive evaluation framework, for both end-to-end and per subtask modes, set up experiment tracking, and followed with several iterations on different components of the document processing pipeline, performing extraction, segmentation, classification, and summarization tasks. The ML team applied various in-context learning approaches for the LLMs and customized Cohere classification models using Convex’ dataset.
The solution was designed to upload a PDF engineering report as input and produce a comprehensive structured summary, which is both machine-readable for downstream tasks, and viewable on the web or exportable as a PDF.
Phase 2: Solution Operationalization and Deployment
Provectus initiated the second phase with discovery sessions that covered Convex’ strategic goals and KPIs. This work included:
- Defining and prioritizing business action items to successfully execute against them
- Assessing the existing solution prototype to identify improvement opportunities
- Evaluating the current UI/UX and outlining the desired UI/UX
- Exploring Convex’ technological landscape, infrastructure, security measures, compliance protocols, and business applications
After defining the scope of phase two, Provectus proceeded to refine the AI adoption strategy and process, to ensure seamless integration of the GenAI solution into Convex’ existing business operations. The ML team started by improving the document processing workflow, quality, and infrastructure.
The workflow for processing risk reports employed Retrieval Augmented Generation (RAG) techniques using Cohere’s LLM. Certain extractions use Cohere’s Classify models which incorporated SME’s feedback. The whole workflow is encapsulated in a Docker container, which is stored in Amazon ECR. Upon file upload, the frontend application initiates an Amazon ECS task for file processing, to transform the resulting JSON file into a summarized report.
The enhanced pipeline for text summarization and information extraction was successfully deployed to production. The ML team implemented robust workflows and components for data capture, model versioning, model monitoring, endpoint updates, and triggers. All elements were integrated with CI/CD tools. The cost-efficiency of the entire deployment was ensured through auto-scaling for model endpoints on AWS.
As a result, Convex received a state-of-the-art text summarization and information extraction solution, powered by generative AI. The solution features a robust end-to-end ML pipeline, which makes it easy to improve the model for processing of newly added types of documents. The solution offers an efficient and convenient UI/UX, complete with essential features for effective use of AI-generated summaries by the SMEs, risk managers, and other roles.

Accelerated Risk Assessment with Generative AI
The impact of generative AI deployed by Provectus was transformative.
By leveraging its new solution, Convex was able to reduce the time to value from a few hours to a mere 10 minutes per survey. This newfound efficiency enables its underwriting team to analyze 100 times more reports, leading to better insights and decisions. The high-quality and consistent summarization, combined with the ability of SMEs to review and edit AI-generated summaries, ensures that no critical detail is overlooked.
The collaboration between Convex and Provectus has set a new industry standard for streamlining insurance underwriting, helping businesses to assess risks and issue policies faster and more accurately. It provides a compelling case for generative AI’s ability to optimize operations and scale business outcomes.
For Convex, the utilization of generative AI is not merely an operational upgrade; it is a strategic realignment that positions their company for exponential growth and long-term success.
Moving Forward
- Learn more about Gen AI’s potential from business and technology perspectives in The CxO Guide to Generative AI: Threats and Opportunities
- Assess the readiness of your organization to adopt Gen AI solutions with Generative AI Readiness Assessment with Provectus
- Develop high-value Generative AI use cases with Generative AI with Provectus and Cohere
CONTACT US
Looking to explore the solution?