Transforming Risk Management in Insurance Underwriting with Generative AI
The client facilitates and scales underwriters’ decision-making, enabling them to accurately assess policy issuance risks using AI-generated summaries of engineering reports
The client is an international specialty insurance company with operations in Bermuda, Europe, the UK, and the US, with over $3B in written premiums. It combines its unparalleled experience and reputation with a legacy-free balance sheet, offering clients a wide range of insurance and reinsurance products, and digital risk management solutions. The company's financial strength is underscored by its excellent ratings from AM Best and Standard & Poors.
Challenge
The client was looking to reinvent its underwriting operations by enabling its underwriters to assess risks and make faster and more accurate decisions about extending or denying policies to potential clients with the help of Generative AI. Previously, its subject matter experts (SMEs) would spend days reviewing 100-page engineering reports of the objects to be insured. With Gen AI, the reports can be summarized in mere minutes, dramatically accelerating policy issuance while balancing risks.
Solution
Provectus developed a state-of-the-art Gen AI solution that automatically summarizes engineering reports, extracting key highlights, such as object information, positive and negative points of interest, and object KPIs. At the core of the solution is Cohere Command, a Large Language Model (LLM) hosted on Amazon Bedrock. Provectus applied the in-context learning approach to perform highly accurate summarization and extraction tasks, specifically tailored to engineering reports in PDF format.
Outcome
The client received a modern solution that can process and analyze engineering reports, and generate summaries that can be either viewed on the web or exported as a PDF. These summaries replicate the elements that its SMEs produce manually, helping underwriters to assess risks and make decisions faster, at scale. The summarization process takes mere minutes per report, enabling the client to insure more objects, reduce the cost of products sold, and increase annual recurring revenue (ARR).
3 weeks
From the project’s inception to a Proof of Concept (PoC) in production
10 min.
From a 100-page engineering report to a comprehensive summary
100x
More objects analyzed and insured, leading to an increase in ARR
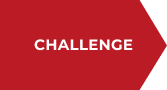
Streamlining Risk Assessment and Policy Issuance in Insurance Underwriting Through AI & Automation
The client is an international specialty insurance company, combines its unparalleled experience and reputation with a legacy-free balance sheet, underscored by its excellent ratings from AM Best and Standard & Poors. By offering the widest selection of insurance, reinsurance, and digital risk management products, it enables its clients to maximize efficiencies and reduce costs, while balancing the risks of operating in a turbulent environment.
The client’s leaders are committed to reinventing the business model through end-to-end digitalization of operations, and by empowering data-driven decision-making for all roles and functions. With new advancements in Generative AI and Large Language Models (LLMs), they saw an opportunity to enhance efficiencies throughout the organization, from Claims and Finance to HR and Portfolio Optimization. The synergy of Generative AI and Data & Analytics is viewed as a pivotal catalyst for the company’s digital transformation.
Insurance underwriting is one area that was strategically prioritized to be augmented by Gen AI and LLM-powered solutions. By leveraging Generative AI, the leadership expected their underwriters to be able to assess risks and make policy issuance decisions more quickly and accurately. They would no longer need to wait for subject matter experts (SMEs) to summarize complex engineering reports of objects, sites, and facilities to be insured.
Previously, before the underwriters could offer a new policy to a potential client, subject matter experts had to manually evaluate 100-page engineering reports that included all technical details of the object. The SMEs would review and summarize these reports, focusing on such key aspects as object information, positive and negative points of interest, and object KPIs. The summarization process was exceedingly time- and resource-consuming, taking days or even weeks before the underwriting team could start their analysis.
Recognizing the need for rapid adoption of Generative AI, the leaders leadership held a competition dubbed the “Hot House” event to select a service provider. Competitors were tasked with developing a solution capable of extracting information from engineering reports in PDF format, and using the extracted data to generate comprehensive summaries.
The “Hot House” judges were a panel of the client’s SMEs and underwriters who performed these tasks daily. After evaluating various Gen AI solutions — each built on tight timelines by multiple contenders — they selected Provectus as their preferred technology provider. The Provectus solution excelled in the accuracy of AI-generated summaries, and overall “technical desirability.”
As a result, the company joined forces with Provectus, an AWS Premier Consulting Partner, to develop and deploy a state-of-the-art report summarization and information extraction solution powered by Generative AI and Cohere’s Large Language Models, hosted on AWS.

Going Beyond Manual Report Summarization with Gen AI and Cohere’s Large Language Models on AWS
Provectus tackled the project in two major phases:
- The first phase centered on developing a Proof of Concept (PoC) for the “Hot House” event. The Provectus team quickly adapted Cohere’s Command Large Language Model (LLM), hosted on Amazon Bedrock, to summarize and extract engineering reports in PDF format. The team collaborated with SMEs and risk engineers to incorporate feedback.
- The second phase focused on operationalizing and deploying the Gen AI solution for real-world use. This included setting up end-to-end ML pipelines, establishing CI/CD processes, implementing model versioning and monitoring, and delivering a user-friendly UI/UX for their underwriters.
Let’s examine these two phases more closely.
Phase 1: PoC Development and “Hot House” Event
To compete in the “Hot House” event, Provectus was tasked to design and build a PoC solution for processing of extensive engineering reports. The solution would extract information about the insured object and classify and summarize the text, highlighting the following key elements:
- Object information (name, location, age, etc.)
- Positive points of interest
- Negative points of interest
- Object KPIs, such as the number of tier 1 and 2 events and the total number of potential losses, including business interruption.
While the process described can be decomposed in a few tasks from the Natural Language Processing (NLP) realm, it was clear that the client had to leverage Generative AI and Large Language Models. This was due to the complexity of the language and domain in the input reports, the volume and size of the reports, the scattered nature of the information to be distilled, and the limited availability of labeled data.
Below is the high-level architecture diagram of the solution proposed by Provectus.
To start development, Provectus requested that the client provide 100 examples of risk engineering reports, along with up to 20 summaries of selected engineering reports that were manually produced by their SMEs and risk engineers.
Provectus rapidly developed the proposed solution using a mix of AWS services, third-party platforms, and open-source frameworks. Central to this solution is Cohere’s Command LLM, accessible via Amazon Bedrock. The Provectus ML team established a comprehensive evaluation framework, for both end-to-end and per subtask modes, set up experiment tracking, and followed with several iterations on different components of the document processing pipeline, performing extraction, segmentation, classification, and summarization tasks. The ML team applied various in-context learning approaches for the LLMs and customized Cohere classification models using the client’s dataset.
The solution was designed to upload a PDF engineering report as input and produce a comprehensive structured summary, which is both machine-readable for downstream tasks, and viewable on the web or exportable as a PDF. As demonstrated below, these summaries include all elements that their underwriters manually create.
The Provectus solution, showcased at the “Hot House” event, excelled in two key metrics according to a panel of judges: the accuracy of AI-generated summaries and overall “technical desirability,” which includes cost-effectiveness, architectural robustness, flexibility, scalability, and time-to-value. By utilizing Cohere’s Command LLM, Provectus not only met but surpassed the evaluation criteria, setting the stage for continued collaboration with the client in the next phase.
Phase 2: Solution Operationalization and Deployment
Provectus initiated the second phase with discovery sessions that covered strategic business goals and KPIs, to define and prioritize action items to successfully execute against them. The team assessed the existing PoC for improvement opportunities, evaluated the current UI/UX, and outlined the desired UI/UX. The client’s technological landscape, infrastructure, security measures, compliance protocols, and business applications were evaluated.
The following were identified as action items in phase two:
- Enhancing the quality of text summarization and information extraction
- Improving the stability and performance of the text summarization and information extraction solution delivered in phase one
- Developing new functional UI and delivering new UX features
- Resolving all identified issues and bugs to achieve “production readiness”
- Implementing essential product and technical analytics tools
- Improving and deploying the solution’s ML model with associated pipelines, workflows, and infrastructure.
After defining the scope of phase two, Provectus proceeded to refine the AI adoption strategy and process, to ensure seamless integration of the Gen AI solution into the client’s existing business operations. The ML team started by improving the document processing workflow and infrastructure.
The workflow for processing the engineering reports was designed, built, and delivered as follows:
- The processing pipeline for an input engineering report was delivered as a combination of key-value extractors, text segmenters, and text classifiers orchestrated by the open-source Python library, spaCy.
- The extraction of key-value elements was implemented as question answering (Q&A) using Retrieval Augmented Generation (RAG). Cohere’s Embeddings LLM is used to convert document chunks into vectors, which are stored in the Chroma vector database. Cohere’s Command LLM is employed to perform question answering over the index to obtain the key-value elements.
- The extraction of negative and positive points of interest was implemented by combining several document chunk classifiers. These are Cohere’s Classify models, trained on embeddings from a points-of-interest dataset. Cohere’s Embeddings LLM was employed to tap into the dataset’s vectors.
- The document processing pipeline is encapsulated in a Docker container, which is stored in Amazon ECR. Upon file upload, the frontend application initiates an Amazon ECS task for file processing, to transform the resulting JSON file into a summarized report.
The enhanced pipeline for text summarization and information extraction was successfully deployed to production. The ML team implemented robust workflows and components for data capture, model versioning, model monitoring, endpoint updates, and triggers. All elements were integrated with CI/CD tools. The cost-efficiency of the entire deployment was ensured through auto-scaling for model endpoints on AWS.
As a result, the client received a state-of-the-art text summarization and information extraction solution, powered by Generative AI. The solution features a robust end-to-end ML pipeline, which makes it easy to improve the model for processing of newly added types of documents. The solution offers an efficient and convenient UI/UX, complete with essential features for effective use of AI-generated summaries by the client’s SMEs, risk managers, and other roles.

Streamlining Insurance Underwriting: Accelerating Risk Assessment and Enhancing Policy Issuance with Gen AI
The insurance industry is characterized by manual, time-consuming processes, particularly when it comes to underwriting. The client’s leadership recognized the transformative potential of Generative AI and Large Language Models to overhaul this critical business function.
The company sought to leverage Generative AI to streamline, accelerate, and scale its underwriting decisions. The challenge lay in the labor-intensive, manual processing of 100-page engineering reports that their SMEs and risk managers would review for days before the underwriting team could make decisions on whether or not to extend a policy to a potential client. That created a bottleneck that slowed down risk assessment, delayed underwriting decisions, and limited the company’s potential for serving high-value enterprise clients.
The goal was clear: to transform an insurance underwriting process that took days into one that could be completed in mere minutes, without compromising the quality of risk assessment.
The company joined forces with Provectus to develop a Generative AI-powered solution that would automatically extract structured information from engineering reports and condense it into comprehensive summaries that include object information, positive and negative points of interest, and object KPIs — all elements that their SMEs once produced manually. The solution allowed the client to not only accelerate the underwriting process, but to also maintain the quality and consistency of report analysis, matching the expertise of seasoned SMEs.
The impact of Gen AI adoption was transformative. By leveraging its new solution, the client was able to reduce the time to value from 10 days to a mere 10 minutes per survey. This newfound efficiency enables its underwriting team to analyze 100 times more reports and insure more objects, with less human intervention. The high-quality and consistent summarization, combined with the ability of SMEs to review and edit AI-generated summaries, ensures that no critical detail is overlooked.
The collaboration between the client and Provectus has set a new industry standard for streamlining insurance underwriting, helping businesses to assess risks and issue policies faster and more accurately. It provides a compelling case for Generative AI’s ability to optimize operations and scale business outcomes. For the client, the utilization of Generative AI is not merely an operational upgrade; it is a strategic realignment that positions their company for exponential growth and long-term success.
Moving Forward
- Learn more about Gen AI’s potential from business and technology perspectives in The CxO Guide to Generative AI: Threats and Opportunities
- Assess the readiness of your organization to adopt Gen AI solutions with Generative AI Readiness Assessment with Provectus
- Develop high-value Generative AI use cases with Generative AI PoC with Provectus and Cohere
CONTACT US
Looking to explore the solution?