Data Governance Practice
at Provectus
In an age when AI is increasingly impacting businesses, data is the currency that keeps everything up and running, much like electricity keeps our cities humming. Unfortunately, getting your organization’s data to power your business operations is not exactly a straightforward process.
Gartner projects that by 2025, an estimated 80% of organizations striving to expand their digital businesses will likely falter unless they adopt a more modern approach to data and analytics governance. This is also a top concern for data leaders: A 2023 survey from MIT CDOIQ shows that 45% of Chief Data Officers (CDOs) view data governance as a key priority.
With a burgeoning rise in generative AI, the ability to properly govern your data end-to-end is becoming ever more important. Without proper data governance in place, your organization may not be able to fully trust the integrity and outputs of your generative AI models.
At Provectus, we recognize the growing demand for data democratization — making data readily accessible throughout the organization — balanced with the imperative of maintaining stringent safety controls, to ensure that data remains secure and stays compliant with evolving regulations.
This white paper takes you through the basics of data governance, its challenges, and how to tackle them, with vivid examples of successful data transformations. We share with you the Provectus approach to data governance, along with our solutions, services, and best practices. Learn how you can take your organization to the next level with Provectus Data Governance.
– Kevin Lewis, Global Practice Principal for data and analytics at AWS Professional Services, “Data Governance Master Class”
While the given definitions do not contradict each other, they do demonstrate how the understanding and implementation of data governance as a practice can vary greatly from one organization to the next.
In all cases, the concept is given broader meaning than just data security or the implementation of policies for working with data and its compliance. These were popular interpretations 5-10 years ago, and they are still a part of data governance, but today’s data governance touches on a wider range of issues.
At Provectus, we believe that the topic of data governance should capture the attention of everyone, from business executives down through the entire organization, including technology roles. We define eight key aspects of data governance to guide and inform this wide-ranging involvement.
The challenges in data governance aren't new. If we glance back to the early 80s when classic data warehouses emerged, many aspects of data governance were already in place. Tools for data modeling, source-to-target matrices, comprehensive documentation, dedicated security departments tasked to ensure compliance through security policies, and the core principle that every data element should have an owner, were all well-established.
As we moved into the late 1990s and early 2000s, the era of Big Data began. This revolution brought with it the capability to process vast volumes of information, albeit less structured and with greater volatility. These systems not only changed the way data was processed, but also democratized it. While managing these vast systems demanded significant effort, it also empowered more companies to adopt a data-driven approach.
With these advancements, new challenges arose, but solutions were also at hand. Some borrowed from conventional application development like logs, metrics, and traces. However, issues associated with the development of data catalogs became increasingly significant, particularly in managing a diverse array of data objects, addressing the rapid and continuous change (velocity) of data, and dealing with the variety and complexity of data types and sources.
The advent of cloud technologies in the late 2000s added another layer of complexity. Advancements in Generative AI with its potential to drive a completely new level of productivity only increased the demand for data governance.
Data processing now faces newfound challenges. Consider these three indicative metrics:
Ratio
Pipelines per Engineer
Journey
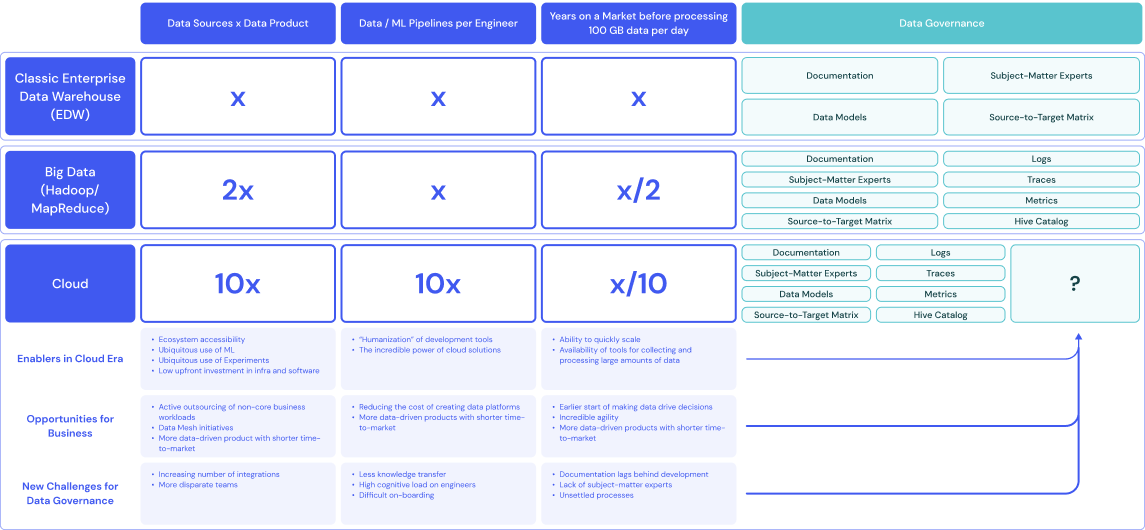
Where does your business stand in terms of data governance adoption? Data governance in any organization does not simply exist; it spans a spectrum of evolution from having no governance measures in place to achieving a comprehensive, highly effective governance framework. You can gauge your organization’s confidence in its data management by addressing the following critical questions:
- How are data assets linked to the business terms we use?
- How are our data models maintained?
- How familiar are we with our data accessibility?
- How effectively do we collaborate on data assets?
- How is data lookup managed?
- How do we monitor PII (Personally Identifiable Information) data?
- How do we ensure the quality of our data?
- How do we trace data lineage?
- Who owns our data?
- How do we categorize and search for our data assets?
- What is our budget allocation for data assets?
If you can answer these questions with confidence, congratulations! You’re likely among the top tier of businesses with impeccable data governance. However, if some questions pose challenges or seem out of context, it’s an indicator that specific areas in your data governance practice might benefit from a closer look and a possible overhaul.
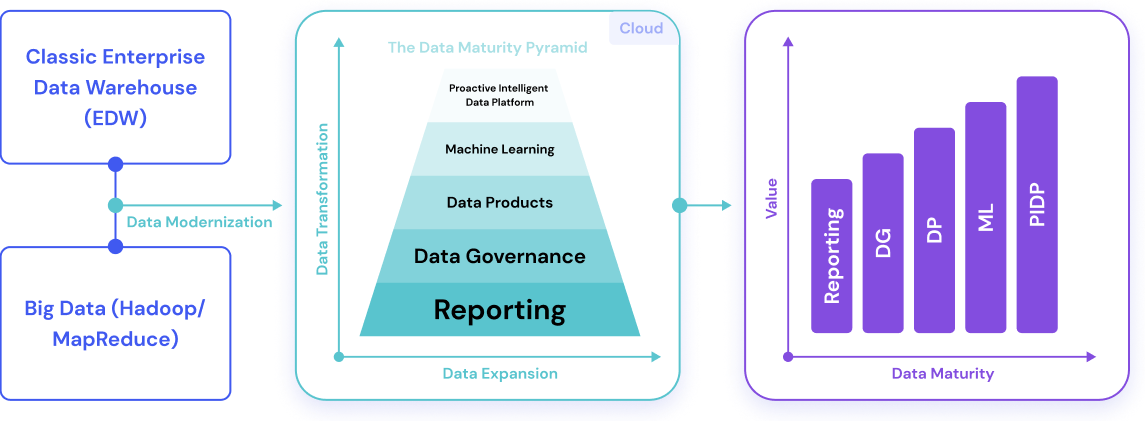
Central to data governance is the concept of Data Strategy. Provectus asserts that any initiative implementing a data strategy falls into one of three distinct categories:
This represents the progression from traditional data warehouses, toward Big Data solutions, to modern cloud-based infrastructures.
This stage involves refining and upgrading your data platform, employing new methodologies, and progressing in your data maturity journey. For more insights on data maturity levels, we invite you to explore our in-depth article.
Expansion is about enhancing the platform’s capabilities, broadening its functions, and growing within the established maturity landscape.
With our rich experience spanning over 13 years, Provectus excels in guiding businesses through these strategic categories. We understand that each type necessitates a unique approach. Modernization, for instance, demands the preservation of data governance standards while migrating to novel solutions and modifying existing practices. Transformation might require the introduction of innovative tools, along with the inception of new processes, reshaping organizational structures, and offering comprehensive training sessions. Expansion concentrates on amplifying features and optimizing workflows.
The core intent of data strategies is to advance through the various stages of data maturity, culminating in a proactive intelligent data platform where business operations and initiatives are efficiently orchestrated by AI under human supervision. This evolution drives smarter, more strategic, and data-driven business operations, featuring key developments such as:
Integrating a holistic, insight-driven, business-focused approach to managing applications and IT across different business verticals, command centers consolidate process and application management, ensuring alignment with business goals.
Offering real-time visibility, decision-making, and execution capabilities concentrated within individual business verticals, Control Towers facilitate end-to-end management within a single business vertical, enhancing efficiency and responsiveness.
Creating digital representations of physical objects, people, or processes, contextualized within a digital version of their environment, Digital Twins aid in simulating real-world situations and outcomes, enabling better decision-making through advanced insights.
When embarking on a data strategy path, data governance is not an option; it is an absolute necessity. Partnering with Provectus makes your journey more navigable and rewarding.
General Considerations:
- Custom Processes: Every organization is unique. Our Data Governance experts strive to design processes that fit each company’s needs after a detailed discovery session.
- Getting Everyone on Board: It is important that main stakeholders agree with and approve of data governance initiatives. Their support helps everything run smoothly.
- Chain of Responsibility: A key part of successful data governance is having a clear line of responsibility, from data owners, to data stewards, to engineers.
For Data Modernization:
- Get Experts in Early: If your organization already has data governance experts, get them involved from the start.
- Training First: Before any changes are implemented, data experts should be trained on what to expect in the future.
- Include Governance in Decisions: Things like data quality, security, and costs should be key considerations in every decision made.
- Keep Up the Good Work: If a mature platform is being modernized, the new system’s governance should be as good as or better than the old one.
For Data Transformation:
- Establishment: Some platforms might not have a clear governance strategy. Data Governance experts need to set one up and make sure it is linked to a potentially impactful business goal.
- Adjusting to Changes and Setting Priorities: If the transformation is more about Machine Learning or Data Products than data governance, two main tasks stand out: making sure governance fits with the new requirements, and identifying the most important aspects of data governance to focus on.
For Data Expansion:
- Add to Governance: As a business grows, governance should grow with it, adding new elements as needed. For instance, introducing data modeling practices where none exist, or building a process for managing infrastructure costs per data product, etc.
- Check and Adjust: Make regular checks, like assessments and creating data governance charts; hold workshops on immersion days with hands-on on best practices on emerging technologies
- More Growth, More Governance: The bigger the data layer becomes, the more governance is needed. Every new business project might need new methods or better ways of doing things to make sure data is handled right.
At Provectus we believe that establishing a clear connection among individuals who set governance policies, those who bring these policies to life on the business side, and those who implement them technologically, is critical.
Our team has years of expertise in rolling out data and AI-driven solutions. We stand ready to guide your journey by assisting in streamlining processes, devising a robust roadmap, charting data strategies, and pinpointing appropriate governance practices to successfully implement business initiatives.
Provectus has a strong track record of developing and deploying solutions across all phases of data strategy, including modernization, transformation, and expansion. Our expertise encompasses five integrated practices, each tailored to deliver specific benefits:
- Data Practice: Aids organizations in effective data management and utilization, helping them make informed, data-driven decisions.
- Machine Learning Practice: Equips businesses with the ability to automate processes, extract insights from data, and create predictive models, enhancing product and customer experiences.
- Data Quality Practice: Ensures the accuracy and reliability of data, helping clients make dependable decisions and avoid potential pitfalls.
- DevOps Practice: Streamlines software development and IT operations, accelerating product delivery without compromising quality.
- General Application Development Practice: Designs and deploys tailor-made applications, allowing businesses to address unique challenges and tap into new opportunities.
Together, these practices have successfully delivered projects across various sectors, for businesses ranging from mid-sized companies to industry leaders.
The Provectus approach to tools is as dynamic as the data landscapes we navigate. We are not biased toward using a specific tool. Our approach is to address business challenges with the most fitting tool at hand.
When we identify gaps in available solutions, we take the initiative to assist our clients in their data strategy pursuits. A shining example of a tool we proudly stand behind is Open Data Discovery (ODD).
Introduced to the Open Source community by Provectus in 2021, Open Data Discovery began its journey as a Discovery tool whose trajectory has been marked by rapid and extensive enhancements. ODD expanded to envelop additional facets of Data Governance, with the integration of Data Lineage, Data Quality, and Data Glossaries capabilities. But our ambitious roadmap for the ODD tool does not stop there. We are geared towards encompassing the entire spectrum of Data Governance practices, with plans to add Data Modeling, Data Security, Data Cost, and Master Data. This developmental path not only captures our vision, but also crystallizes our rich experience of working on intricate data-driven projects.
Use case: When migrating from a classic data warehouse, organizations face the challenge of transitioning from traditional documentation methods, such as Source-to-Target Matrix, to more dynamic and modern systems. Open Data Discovery (ODD) can streamline this process by efficiently managing data transformations in the modern stack.
Processes:
- Data Assessment: Before initiating any migration, it is imperative to assess the current state of the data in a classic data warehouse, and identify the transformations, dependencies, and relationships documented in the Source-to-Target Matrix.
- Mapping & Migration Strategy: Designing a roadmap for migrating data transformations from a classic warehouse to a modern platform. Determining which transformations will be handled natively by the modern stack, and which will be managed by ODD.
- Integration with ODD: Begins by setting up Open Data Discovery to integrate with the modern stack. This allows for a smoother transition of transformations from the classic data warehouse.
- Validation & Quality Check: Once data is migrated, its integrity must be validated. Ensure that transformations managed by ODD match their intended results in the modern stack, mirroring the original processes in the classic warehouse.
- Feedback Loop: Establish a feedback mechanism for users to report any inconsistencies or issues they encounter post-migration. This ensures continuous improvements in the migration process.
People:
- Data Architects: Guide the data modeling and structural decisions made during the migration process.
- Data Engineers: Responsible for the actual migration of data transformations from the classic warehouse to the modern stack.
- Data Governance Team: Ensures that the data adheres to organizational policies and quality standards, both pre- and post-migration.
- DevOps and IT Support: Assist in integrating ODD with the modern stack and troubleshooting any technical challenges that arise.
- End-users/Stakeholders: User feedback is invaluable in identifying any potential issues after migration, and ensuring that business needs continue to be met.
Tools:
- Open Data Discovery (ODD): Serves as the main tool to manage data transformations in the modern stack, providing a comprehensive solution for data governance, lineage, quality, and more.
- Modern Data Platform Tools: Tools may vary depending on the modern stack being used. Examples could be cloud platforms like AWS, Microsoft Azure, or GCP, and modern data warehouse solutions like Snowflake or Redshift.
Use case: Implementation of a RAG (Retrieval Augmented Generation) system that allows for navigation through corporate data using natural language, and for the creation of proactive systems that respond to risks or opportunities that emerge in the company’s operational activities.
Processes:
- Data Inventory & Categorization: Begin with a comprehensive audit of all available data, tagging and categorizing it according to relevance, sensitivity, and application.
- Data Integration: Streamline processes to ensure data from diverse sources is integrated cohesively, reducing silos.
- Data Quality Assessment: Continuously monitor and validate the data to maintain its accuracy and reliability.
- Data Governance Strategy Implementation: Establish a framework to guide the deployment and continuous adaptation of the RAG system, ensuring adherence to data governance principles.
- Training and Feedback Loop: Train the RAG system with a diverse range of queries, incorporating feedback from users to refine its performance over time.
People:
- Data Stewards: Oversee data quality and categorization, ensuring data consistency and relevance.
- Data Engineers: Handle the integration, processing, and transformation of raw data into structured datasets.
- AI Specialists: Design, train, and optimize the RAG system to deliver the desired outcomes.
- End-Users: Regular users provide feedback on the RAG system’s performance and efficiency, which is essential for iterative refinement.
Tools:
- Open Data Discovery (ODD): Assists in data discovery, lineage, and quality checks, providing a unified view of the data landscape.
- Feedback Collection Platforms: Tools that allow users to provide feedback on system performance, aiding in iteratively refining the system.
Use case: Implementation of an abstract feature store for simplified navigation through corporate data for building Machine Learning Applications.
Processes:
- Identification of Data Assets: Begin by understanding and cataloging existing data assets that can be instrumental in the feature store.
- Data Validation & Cleaning: Ensure that data entering the feature store is of high quality and free from discrepancies.
- Feature Engineering: Extract and create essential features from raw data sources that will be valuable for ML applications.
- Metadata Annotation: Describe each feature’s origin, purpose, and other metadata to make it easily discoverable.
- Version Control: Implement a system to track changes and versions of the features over time.
- Integration with ML Pipelines: Ensure that the feature store can feed data seamlessly into ML workflows and pipelines.
- Monitoring & Maintenance: Regularly monitor the feature store’s performance and data quality, making updates as necessary.
People:
- Data Engineers: Responsible for extracting, transforming, and loading data into the feature store.
- Data Scientists: Play a key role in feature engineering and determining which data attributes are essential for ML models.
- MLOps Engineers: Ensure that the feature store integrates well with ML pipelines and deployment processes.
- Data Stewards: Oversee the quality, security, and usability of the data in the feature store.
- Business Analysts: Offer insights on which features might be relevant from a business perspective.
- DevOps and IT Support: Provide support in terms of infrastructure setup and scalability considerations.
Tools:
- Open Data Discovery (ODD): Given its capabilities in data governance, ODD can play a role in identifying and cataloging features.
- MLOps Platforms: Platforms like MLflow, Kubeflow or Provectus MLOps Platform for integrating the feature store with ML workflows.
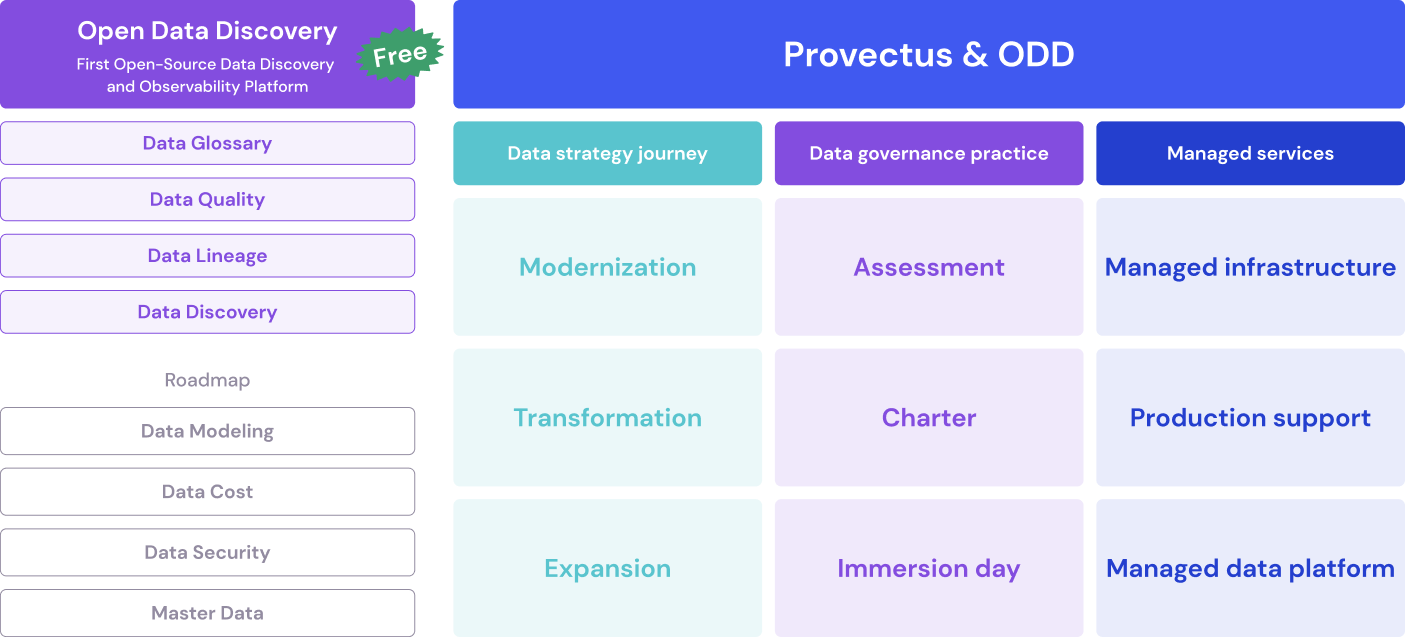
The Provectus approach to tackling data governance challenges is depicted in this framework. Our expertise in implementing business-driven data strategy initiatives encompasses data modernization, transformation, and expansion. For each of these initiatives, we integrate data governance practices to ensure clarity, security, and efficiency. Our dedicated data governance team is available to assist organizations in implementing and maintaining data governance activities. We have created a distinct tool designed to cover every technical facet of data governance. We provide managed services to ensure that any data strategy is efficiently initiated and maintained.
Provectus offers a comprehensive solution for all your data governance needs. Here is an overview of our tailored solutions.
Data Modernization
Transitioning data platforms to the cloud is our forte, with an impressive portfolio of successful migrations and deep expertise in cloud integrations. Among our offerings are:
- Migration Acceleration for AIML on AWS: Move your data and applications to AWS, to start capitalizing on the massive potential of AIML for your business. More details about the program
- Amazon EMR Migration: Optimize your Big Data Solution on Apache Hadoop/Spark by migrating to Amazon EMR. Learn more
- Apache Kafka Migration to Amazon MSK: Enhance your streaming data processing by migrating to Amazon MSK. Explore here
Data Transformation
We stand at the forefront of Generative AI technologies, offering cutting-edge solutions for businesses ready to take the next data leap.
- Generative AI Guide: Delve into The CxO Guide to Generative AI: Threats and Opportunities. Read here
- Analytics & Decision Intelligence: Provectus is honored as a Representative Vendor in the Gartner Market Guide for A&DI Platforms in Supply Chain. Learn More
- AI Transformation: Stay updated with the latest in Machine Learning and reshape your business strategy. Discover more
Data Expansion
Expand and enrich your data landscape with our specialized solutions.
- AI Solutions: Implement industry-specific AI solutions to drive value. Explore here
- MLOps Platform: Optimize ML model delivery from prototype to production. Learn more
- Data Quality Assurance: Ensure the highest data quality for precise analytics and decision-making. More details
Data Governance Assessment
We begin with a thorough analysis of your existing data governance landscape, pinpointing strengths, gaps, and opportunities. Our assessment serves as a foundation for designing strategic improvements.
Data Governance Charter
Our charter is a blueprint for effective data governance, delineating roles, principles, and decision-making frameworks. It provides a roadmap to aligned, transparent, and efficient data governance.
Data Governance Immersion Day
Experience the intricacies of data governance firsthand. This immersive day provides a practical dive into data governance, fostering collaboration, understanding, and strategy-building.
Discover our range of managed services tailored to foster business growth and innovation. Visit our offerings
Managed Infrastructure
Our service simplifies cloud infrastructure management for your applications, ensuring top-notch performance, security, and cost-efficiency.
Production Support
We ensure optimal performance and reliability for your applications in production environments, minimizing potential disruptions.
Managed Data Platform
Our service streamlines the management of your data platform, guaranteeing unparalleled performance, robust security, and economical solutions.
With our suite of solutions, Provectus is poised to partner with businesses at every stage of their data journey, ensuring success, growth, and innovation.
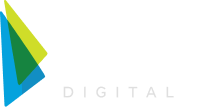
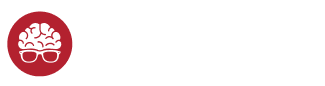
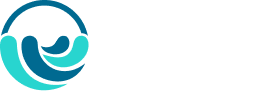
Ready to get started with your Data Governance journey?
We hope that this white has enhanced your understanding of data governance. We have defined data governance, outlined ongoing challenges and potential solutions, and shown how it should fit into the bigger picture of your organization.
Data governance provides the guardrails that keep your data accurate, secure, compliant, and easy to use — key qualities that every data- and analytics-driven business needs to succeed and stay customer-focused. We have looked at processes, people, and technology, and shared insights about their roles in a data governance strategy. We have also shared Provectus’s vision of data governance.
Data governance is the most important differentiator of success in a world of ubiquitous AI. With the right approach, your organization can protect and control its data, and turn it into a source of strategic value.
See the Provectus privacy policy for details on how we collect, use, and share information about you.