Reimagining Insurance with Generative AI:
The Ultimate Guide for Business Leaders
In the span of just a few years, generative artificial intelligence (GenAI) has transitioned from just another concept in AI research to a major driver of business value. Unlike traditional AI, GenAI can create unique outputs — from text, images, audio and video, to code and data — through simple human commands executed within seconds. This revolutionary shift has spurred organizations in industries as diverse as banking, healthcare, and manufacturing to invest in GenAI for improved customer interactions, enhanced operational efficiency, and unprecedented product innovation.
Generative AI is quickly becoming the new normal in the insurance industry. Nationwide is exploring the use of GenAI as an assistant for customer service representatives. The GenAI platform analyzes customer calls in real time, providing prompts and data to help representatives resolve issues swiftly and improve the customer experience. HDI Global is utilizing its proprietary GenAI system, HDI-GPT, to extract real-time insights from unstructured data, be it text or images. GenAI assists the staff in interpreting legal contract terms and conditions through content summarization and real-time assessment of insurance claims.
The potential of generative AI in insurance is immense. According to estimates from the McKinsey Global Institute, insurance organizations are projected to be one of major beneficiaries of GenAI, with an annual potential of $50B to $70B. This represents about 3% of the total industry revenue, with increases driven by better operational efficiency and the automation of micro-tasks such as claims processing and underwriting. GenAI use cases in customer-facing operations, marketing and sales, software engineering, and risk and compliance are also cited as major value drivers.
For the insurance industry, adopting generative AI means more than keeping up with technology trends. It is about making a strategic choice to innovate beyond traditional AI to remain competitive. Tried-and-true AI use cases such as predictive analytics, anomaly detection, and automated document processing can now be complemented by GenAI solutions for hyperpersonalized customer service and insurance advice; intelligent document search, summarization, and generation; and custom knowledge agents for business teams. With generative AI, insurance leaders have a once-in-a-lifetime opportunity to redefine their business.
This guide offers C-level executives in insurance a clear, actionable roadmap for adopting generative AI. From understanding basic principles and potential applications to tackling the complexities of building their first solution, this Guide will help executives to navigate the GenAI landscape, to drive growth, efficiency, and innovation.






- Marketing and sales
- Customer operations
- Risk and compliance
- Software engineering
- Strategy and finance
- Supply chain and operations
- Corporate IT and product R&D
- Talent organization
In practice, “horizontal” GenAI use cases empower a variety of applications, including dialogue generation for virtual assistants, automated code generation, personalized messaging for marketing and sales, and much more.
However, the insurance industry has specific requirements. “Vertical” GenAI use cases — the ones that are organized by unique and more focused, sector-specific tasks — present more opportunities to create sustainable and measurable value. But these opportunities require domain knowledge, contextual understanding, and expertise, often necessitating the fine-tuning of existing foundation models. Some “vertical” GenAI use cases include:
Both horizontal and vertical use cases are more likely to realize at-scale business value for an organization if they are used holistically; that is, if they are seamlessly integrated with other systems, and into processes and teams.
Considering that the insurance industry holds vast amounts of sensitive customer data, it is crucial to strike the right balance between harnessing value from generative AI and managing the associated risks, like hallucinations, biases, phishing, and prompt injections. Consider also the potential for long-term vs. short-term profitability and growth, cost savings, the balance between impact and investment, and the time to achieve measurable impact.
1
2
3
1
The use case discovery phase begins by identifying the problem and a specific action that the organization wants to achieve. Different use cases require various types of foundation models, and it is essential to establish clear goals and metrics from the start. These will direct the selection of the most appropriate model type, as well as the model’s performance, knowledge and understanding levels, latency, and required security measures.
GenAI action to complete:
- Text generation
- Text summarization
- Text translation
- Text classification
- Information retrieval
- Question answering
- Sentiment analysis
- Vector search
2
Foundation models differ not only in type but also in size, which is primarily determined by the number of parameters they contain. In general, large models provide robust performance and offer superior reasoning abilities at increased cost. Smaller, more specialized models are more affordable and easy to deploy, yet they can be challenging to train, maintain, and scale across numerous GenAI use cases.
Foundation model size factors to consider:
- Supporting architecture
- Training data (volume, variety, quality)
- Optimization technique (e.g. quantization)
- Transformer efficiencies
- Choice of learning frameworks
- Model compression techniques
- Cost of inference (run-time)
- Environmental impact
3
Selecting a foundation model for a GenAI use case should be a forward-thinking process. Organizations should consider the immediate task and its potential scalability organization-wide — if such scalability is feasible. Given the specifics of “vertical” GenAI use cases, insurance organizations should understand the implications of scaling on costs, performance and ROI, while also balancing it with GenAI experimentation to find a better FM.
Scaling components to pay attention to:
- Data residency requirements
- Estimates of the traffic volume that will be served
- Talent: upskilling, recruitment, or both
- Costs: minimal up-front investment vs. significant spend down the road
- Operations: in-house vs. managed AI service
Streamlining Insurance Underwriting with Generative AI
Challenge



Solution
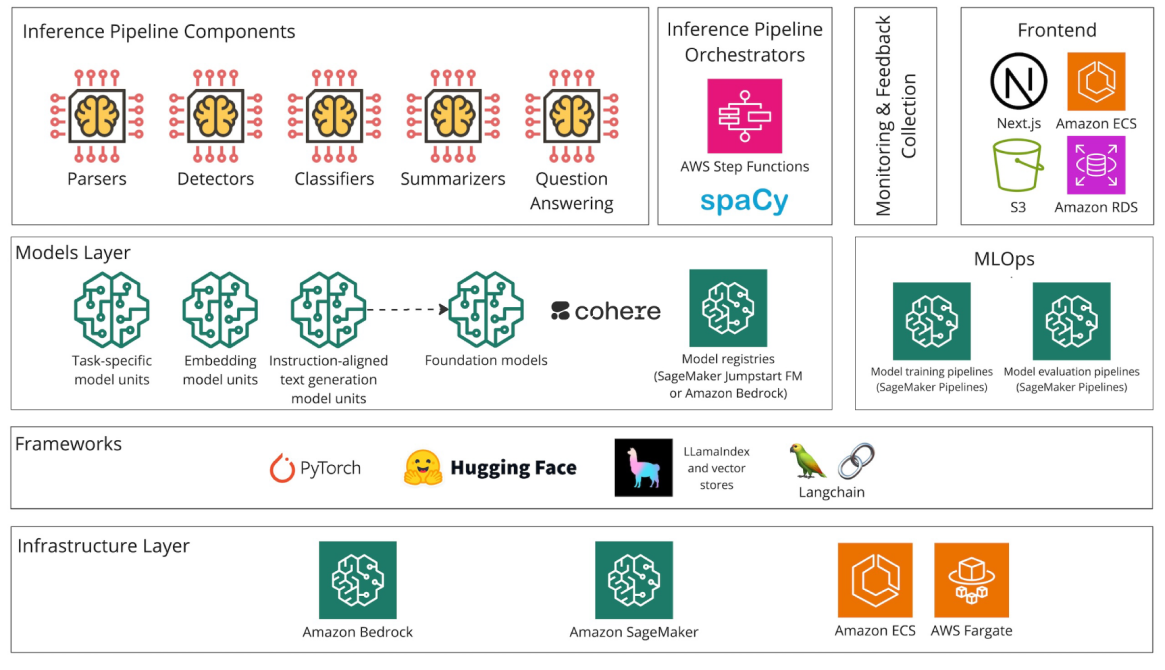
Outcome



Generative AI Adoption Journey with Provectus and Cohere
At Provectus, we believe that a generative AI use case should serve as the essential first step for any company looking to adopt and scale generative AI, while realizing business outcomes. Provectus’ subject matter experts and technical team, in close collaboration with Cohere, have developed an approach to help enterprises quickly adopt the most impactful GenAI use cases. It encompasses two phases:
Our approach is designed to encourage cross-functional collaboration between C-suite Executives, Business Unit Leaders, Data and AI/ML Leaders, and IT professionals. The goal is to streamline the adoption process and effectively measure success criteria.
Deliverables:
- Data and infrastructure discovery
- Prototype of the selected use case deployed on AWS
- GenAI use case prioritization and adoption roadmap
- Solution architecture documentation
- Expected business value and success criteria research
- Detailed plan to deploy to production
- Estimation of use case complexity and risks
- TCO estimates for AWS and FM services
Generative AI is transforming industries rapidly, and leaders in insurance need to closely monitor this technology, poised to add value of between $50B and $70B annually from its adoption. Generative AI presents a prime opportunity to compete in a highly dynamic market where InsurTech startups are challenging the dominance of established enterprises.
However, generative AI adoption is not always straightforward. It necessitates comprehensive organizational changes business- and technology-wise, starting from the adoption of the first GenAI use case, to scaling the technology across various processes and functions. Provectus is ready to support your insurance organization as a valuable partner in your GenAI adoption journey.
Leveraging its deep expertise in the AI domain, Provectus excels in end-to-end AI transformations for enterprises, and in implementing AI solutions on a use-case basis. With pre-built GenAI solutions, Provectus offers its customers risk-free Prototypes, ready to go live within weeks so you can quickly evaluate the impact of generative AI on your operations.
Provectus pioneers the adoption of generative AI technologies with AWS. As an AWS Premier Tier Services Partner with the AWS Machine Learning Competency, AWS Data & Analytics Competency, AWS DevOps Competency, and AWS Migration Competency designations, Provectus is one of the few partners to add AWS Generative AI Competency to its list of specialized credentials.
Our strategic alignment with AWS extends beyond technological advancements. To drive the adoption of generative AI, AWS works closely with Provectus and other partners to fund their customers’ projects. Given AWS’s strong focus on the banking, finance, and insurance industries, insurance organizations are well-placed to receive funding, to help mitigate the costs associated with developing, adopting, and maintaining GenAI solutions.
To address the existing and potential risks of GenAI adoption for its customers, Provectus has launched The AI Landing Zone accelerator, designed to help enterprises take their first steps toward the application of generative AI for real-world use cases while keeping potential risks in check.
From day one, Provectus has stayed true to its mission to help businesses reimagine the way they operate, compete, and deliver customer value with AI. Generative AI marks an exciting new chapter for Provectus, creating unprecedented opportunities for our customers. We encourage all forward-thinking executives to explore, adopt, and leverage generative AI to solidify the future success of their businesses.
See the Provectus privacy policy for details on how we collect, use, and share information about you.