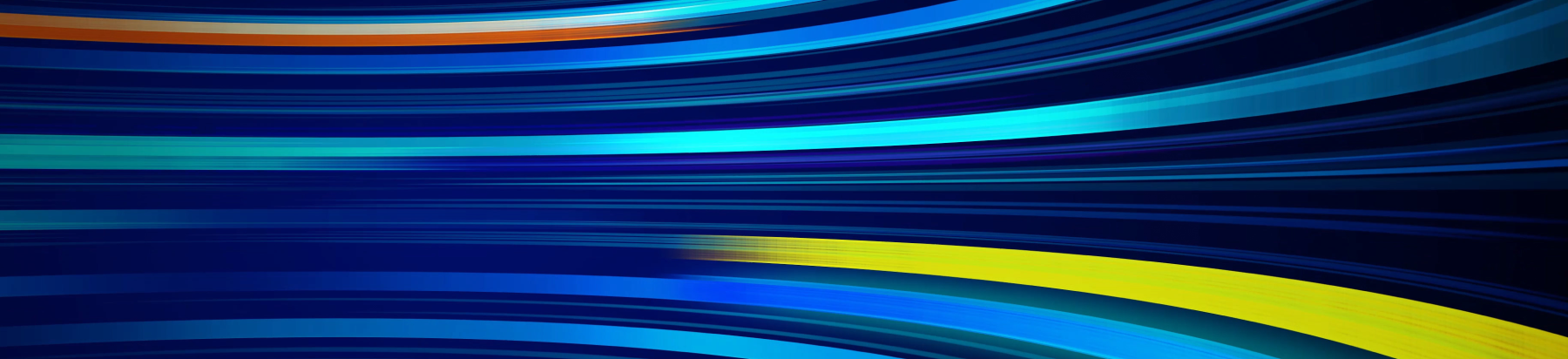
April 5, 2024
11 min read
Shaping the Future of Life Sciences: Innovations and Efficiencies Driven by Generative AI on AWS
Authors:
Matt Ewalt, Executive General Manager, Practice Head – Healthcare & Life Sciences, Provectus
Rinat Gareev, Senior Solutions Architect, Provectus
Rinat Akhmetov, ML Solutions Architect, Provectus
Today the life sciences industry is facing an era of unprecedented challenges — and opportunities — that have the potential to redefine the landscape of biomedical innovation. According to the Life Sciences Market Report 2024, the global life sciences industry is projected to exceed $137 billion by 2028. The sector is at a critical juncture where embracing technological advancements is not just an option, but a necessity for sustaining growth and remaining competitive.
Key challenges in the industry include the escalating cost and duration of drug development, which, according to Deloitte’s Seize the Digital Momentum research, is estimated at $2.2 billion and over a decade per drug. The industry also grapples with the complexity of managing vast and diverse datasets that are essential for breakthroughs in personalized medicine, genomic research, clinical trials, and more. These datasets, often siloed and unstructured, require advanced data analytics and AI/ML solutions capable of extracting value for organizations.
Generative AI, particularly Large Language Models (LLMs), offers a solution to these and other challenges. It holds the potential to revolutionize drug discovery by shortening development and testing timelines, reducing costs by optimizing operations and processes, and enhancing the precision of therapeutic interventions and their post-procedural analysis. For instance, incorporating AI in a clinical trial supply planning system can reduce waste by roughly 50%, with total waste reduced from 70% to 25%.
In the intricate domain of regulatory compliance, specifically in adhering to FDA guidelines, generative AI can make a significant difference. The industry faces a critical need to streamline the processing of various compliance documents and reports across different clinical, manufacturing, and supply chain workflows. Generative AI’s ability to retrieve, process, summarize, and analyze complex regulatory data can reduce time-to-compliance, a crucial factor in accelerating a drug’s journey from lab to market.
In this article, we explore how generative AI can propel the life sciences industry towards a future where agility, precision, and innovation converge to redefine healthcare outcomes. Specifically, we zoom in on one of the most vital applications of generative AI in life sciences — enhancing compliance efficiency through faster at-scale processing of FDA Form 483 Observations using AWS.
Generative AI in Life Sciences: Potential use cases and applications
Life science organizations are facing tremendous pressure to innovate. Following the disruptions of the Covid-19 pandemic, the industry has experienced an explosion of digital data. According to research by RBC Capital Markets, “Today, approximately 30% of the world’s data volume is generated by the healthcare industry. By 2025, the compound annual growth rate of data for healthcare will reach 36%. That’s 6% faster than manufacturing, 10% faster than financial services, and 11% faster than media & entertainment.”
This data is increasingly diverse and complex. It includes clinical study reports and regulatory compliance documents in PDF and other formats, complex clinical trial data and patient records in disjointed Electronic Health Record (EHR) systems and databases, along with genetic, image, microscopy, and other miscellaneous data, such as emails and web content.
The sheer volume, complexity, and diversity of data, coupled with the myriad functions, roles, and operations associated with data processing, management, and utilization, create a vast universe of use cases for generative AI.
- Protein Engineering: Traditional bioengineering methods that involve laborious trial-and-error can be augmented by AI techniques that can rapidly generate and screen protein sequences. Generative AI can help accelerate the process of discovering novel functional proteins with desired properties, such as binding affinity, stability, and immunogenicity. It is crucial for developing new enzymes, therapeutic antibodies, and biomarkers, faster and more efficiently.
- Synthetic Biology on Demand: Generative AI models like ProteinGAN are opening new possibilities for designing genes and pathways that do not exist in nature. This includes applications in biosensor design, biomanufacturing, metabolic engineering, and gene therapy. The use of generative AI democratizes access to synthetic biology, allowing for more rapid and diverse applications, such as creating more efficient enzymes for specific industrial processes.
- Medical Imaging Enhancement: Generative AI can be used to denoise images, increase image resolution beyond the hardware’s limitations, delineate anatomical structures, reconstruct 3D anatomy from limited data, and convert between different imaging modalities. These advancements not only improve the quality of diagnostic imaging, but also make older imaging data more usable for modern research, providing more samples for discovery.
- Simulating Patients and Trials: Generative AI can create diverse simulated patient populations and trials, which is useful in healthcare AI where robust models are needed but patient data can be challenging to access. This includes generating synthetic patient records and simulating virtual clinical trials. Such applications allow for more efficient testing of clinical decisions and discovery, and contribute to the development of precision medicine and wellness applications.
- Scaling Document Operations: In life sciences, information retrieval and summarization are at the core of most document-centric workflows. They form the backbone of decision-making in drug development, clinical trials, and therapeutic strategies, among others. Generative AI’s ability to swiftly navigate, process, and understand vast volumes of document data transforms how human teams access, interpret, and utilize information, scaling document-centric operations across an entire organization.
The examples provided are just a few of the major directions generative AI could go in life sciences. Its use extends even further, spanning various domains such as research and early discovery, clinical development, operations, commercial sectors, and medical affairs. The range of tasks it encompasses includes content generation, code creation, knowledge bases, regulatory intelligence, and more.
At Provectus, we are confident that generative AI has the potential to significantly expedite and enhance the quality of compliance document processing. Drawing from our experience with PSC Biotech, a global life sciences consultancy, we have observed that generative AI can accelerate document-centric operations by 90%, reduce document processing costs by 44%, and increase document throughput tenfold. The adoption of generative AI has led to a substantial acceleration in time-to-compliance, saving 5,000 man-hours per year, and enabling PSC Biotech to achieve an estimated return on investment (ROI) of 93% over 12 months.
Building a generative AI-powered compliance document processing solution on AWS
Life sciences companies are navigating a complex landscape, striving to achieve business goals while adhering to stringent regulatory compliance. Compliance audits and inspections entail significant costs, but the repercussions of non-compliance are far more severe and can include hefty fines and reputational damage, and can negatively impact patient health and safety.
Currently, compliance document processing in life sciences predominantly involves manual procedures. However, the field is undergoing a significant transformation, marked by a shift towards digitalization and automation.
Consider the processing of FDA Form 483 Observations. This document is issued by FDA inspectors after an inspection if they observe conditions that may constitute violations of the Food Drug and Cosmetic Act and related Acts. It records objectionable conditions related to the production and handling of food, drugs, medical devices, or cosmetics, which may lead to product adulteration or harm to public health.
Failure to address issues cited in Form 483 can lead to severe repercussions. The FDA can enforce injunctions, and impose criminal and civil penalties against individuals and companies, such as fines, product recalls, and even arrests. Penalties vary, starting with a warning letter and escalating to fines of up to $100,000 per misdemeanor violation, with potentially higher fines for felony offenses and convictions.
PSC Biotech has long depended on its established manual pipelines to process thousands of complex FDA Form 483 observations (see examples in Figure 1) for clients. Although the document mappers and reviewers have been thorough, the need to automate document processing pipelines is clear. Manual processes lead to rising operational costs, limited throughput rates, inconsistent processing accuracy, and a heightened risk of human errors.
With generative AI, we are confident that compliance document processing, as exemplified by the processing of FDA Form 483 Observations in PSC Biotech’s pipelines, can be significantly streamlined and accelerated. Generative AI can enable human teams to concentrate more on value-adding tasks, such as reviewing the results of AI’s work rather than processing every document in their pipeline, end-to-end.
We explored multiple options to equip PSC Biotech with a generative AI-powered solution capable of extracting and classifying information from observations with high accuracy. Our discovery included a comparative review of various transformer-based encoder models (like BERT), and deep learning and natural language processing (NLP) algorithms. Ultimately, the solution delivered for observation classification was a multi-label classification model capable of categorizing FDA Form 483 Observations across more than 100 labels, while achieving precision and recall rates of 70% or higher. Observations were automatically labeled, facilitating their categorization into various groups. The solution enabled document mappers and reviewers to search for observations by selecting from the model-generated labels. The high-level architecture of this solution is shown in Figure 2 below.
Following is a brief overview of some of the services used for building the solution.
Amazon CloudFront was utilized to deliver the user interface, while Amazon RDS served to store document- and model-related data. User authentication and resource access control were managed by Amazon Cognito. The backend was implemented using Amazon API Gateway, AWS Lambda, Amazon SQS, and Amazon DynamoDB.
The backend launches the document processing pipeline that starts with Amazon Textract as an Optical Character Recognition (OCR) engine, to efficiently process PDF documents. Then, OCR outputs are restructured into a set of extraction candidates: for every candidate, features are generated using the BERT embedding model; featurized candidates are fed into the custom classifier model. These models are deployed using Amazon SageMaker Serverless Inference. AWS Step Functions effectively orchestrates these services, streamlining the building and updating of applications. Amazon S3 was used for intermediate storage of texts, document observations, and model predictions.
The MLOps infrastructure based on Amazon SageMaker was designed and built to facilitate the establishment of pipelines for CI/CD, logging, monitoring, and model retraining. This pipeline was proficient in retraining the existing model and deploying it to production, once improvements in precision and recall were achieved. This setup ensured a seamless and efficient update process, maintaining high standards of model performance in the production environment.
The rapid advancement of generative AI in recent months could further improve our solution for compliance document processing using Amazon Bedrock, a fully managed service that provides foundation models (FMs) from leading AI companies, such as AI21 Labs, Anthropic, Cohere, Meta, Stability AI, and Amazon itself.
Our solution is engineered to seamlessly integrate with the model invocation API of Amazon Bedrock. This ensures that our ability to extract features from FDA Form 483 Observations is not only easily modifiable and adaptable, but also primed to harness the advanced capabilities of any foundation model out of the box. The models available through Amazon Bedrock in particular offer a rich set of functionalities that enable our solution to capture a more comprehensive set of nuances within the regulatory text. This enables our multi-label classification model to categorize observations with unparalleled precision.
By leveraging the advanced capabilities of Amazon Bedrock foundation models, our solution can enhance the labeling automation process, allowing us to not only meet but surpass our initial benchmarks in precision and recall rates — from approximately 70% to as high as 95%.
This approach makes it easy to continually refine the categorization process and equip document mappers with even more sophisticated search functionalities, ensuring that our generative AI solution remains at the forefront of technology in regulatory document processing.
Amazon Bedrock, as well as the entire ecosystem of AWS services, is a viable option for all life sciences organizations that are looking to explore and innovate in the generative AI domain without constraints. AWS democratizes generative AI, helping organizations quickly discover solutions that fit their unique business needs and requirements.
Democratization of generative AI on AWS
According to a Bloomberg Intelligence report, the generative AI market is expected to experience significant growth. From a valuation of approximately $40 billion in 2022, the market is projected to expand to a staggering $1.3 trillion over the next decade.
Despite generative AI’s potential for growth, navigating the generative AI hype remains a challenge. Many companies consider adopting generative AI solutions and experimenting with specific use cases, yet find the prospect of scaling these solutions across the organization daunting. Challenges impeding progress range from issues like custom data availability and data security, to the cost-efficiency and flexibility of foundation models. Concerns about losing intellectual property (IP) to generative AI providers further complicate the situation, making full-scale, unimpeded adoption a complex task for many organizations.
AWS stands uniquely positioned to tackle the challenges of balancing generative AI adoption with generative AI experimentation. AWS offers enterprise-grade security and privacy, access to leading foundation models, and services powered by generative AI. This enables easy building and scaling of generative AI solutions, tailored to specific data, use cases, and customer needs. Organizations ranging from startups to enterprises can assuredly rely on AWS for innovation in generative AI.
AWS’s generative AI strategy rests on four major pillars:
- Simplifying the building and scaling of generative AI applications on Amazon Bedrock with built-in security and privacy.
- Offering the most efficient, cost-effective infrastructure for generative AI, allowing customers to train their models and run large-scale inference on advanced GPUs, custom silicon instances, and Amazon Sagemaker.
- Providing multiple generative AI-powered enterprise applications, enhancing software developer productivity (Amazon CodeWhisperer), supporting data-driven decision-making (Generative BI for Amazon QuickSight), and other use cases.
- Enabling data as the differentiator to customize foundation models and make those models experts on the customers’ specific business, data, and company.
These unique advantages, along with AWS’s varied infrastructure and framework options, provide businesses with multiple entry points and pathways, democratizing generative AI for all. Most importantly, this versatility allows companies to justify moving away from Proof-of-Concept (POC) projects toward real-world applications, powered by generative AI from their inception.
Conclusion
Today life sciences enterprises are increasingly adopting generative AI, striving to balance experimentation with high ROI. They prioritize addressing such critical aspects as governance and compliance, legal and privacy issues, and risk management, to ensure the responsible and successful adoption of AI.
With pre-built generative AI solutions, Provectus offers its customers risk-free POCs and deploys them live within weeks, enabling companies to quickly evaluate the impact of generative AI on their operations.
The AI Landing Zone accelerator by Provectus is designed to help enterprises take their first steps toward generative AI adoption while keeping potential risks in check. From day one, Provectus has stayed true to its mission and is ready to help businesses reimagine the way they operate, compete, and deliver customer value with AI.